Impact of Crop Production on Economic Growth in Nigeria, 1981 – 2018
Joseph M. IBBIH1, Jorji Akudo NWOGU2
1Department of Economics, Faculty of Social Sciences, Nassarawa State University, Keffi Nigeria
2Department of Economics, Federal College of Education (Technical) Gusau, Zamfara State, Nigeria
ABSTRACT: This study examined the impact of crop production on economic growth in Nigeria utilizing annual time series data from 1981 to 2018. The study used Johansen cointegration test, Vector Error Correction test, the impulse response functions and variance decomposition for data analysis after the data series were checked against unit root problem using the Augmented Dickey Fuller unit root test. The study tested and found that increase in the production of cassava, cocoyam, groundnut, guinea corn, maize, millet, rice and yam spurs economic growth in Nigeria in the long-run except for cotton production and with only groundnut production contributing significantly to the output of the Nigerian economy in the short- run. The study, therefore recommends that the Nigerian government should encourage farmers in the production of crops through supply of fertilizer to increase their productivity and food output while improving their use of modern seedlings and technology in the production that may in turn increase the crop production while farmers should be encouraged to adapt best practices in farming to maximize crop yield.
- INTRODUCTION
A review of the agricultural sector and particularly crop production vis-a-vis its contribution to Gross Domestic Product (GDP), export earnings and employment reveals the prominence of the sector in the economies of most African countries. For the continent as a whole, the agricultural sector accounts for approximately 60 percent of total employment, 20 percent of total exports and at least 15 percent of GDP (African Union, 2006). With varying commitment to agricultural development by the African countries, there have been some pockets of success in areas such as; New Rice for Africa (NERICA), and introduction of high yielding cassava varieties for crop production.
Crop production has been practiced since creation to the present era but it has most often been ignored. The oil and gas sectors have taken the major interest with few or less interest place on crop production and agricultural sector generally. Statistics have shown that crop production recorded 8.8% (₦12.82 billion) and 17.1% (₦101.65 billion) in contribution to the nominal GDP in 1981 and 1991 respectively; which is relatively less than its contribution prior to 1970s (CBN, 2015). Crop production in Nigeria has exhibited an upward trend in contribution to GDP over time. According to CBN (2015), crop production was ₦377.31billion (21.4%) in 1994 which increased to ₦1,133.39 billion (24.70%) and ₦4,419.06 billion (25.51%) in 1998 and 2004 respectively. The performance of crop production in Nigeria has been trending upward with recent decline in its contribution to GDP to the tune of ₦11,683.9 billion (21.39%), ₦17,189.97 billion (18.26%), and ₦21,096.11 billion (18.55%) in 2010, 2015 and 2017 respectively. However, the nominal output of crop production has exhibited upward trending pattern in terms of performance from 1981 to 2017. Generally, crop production is the hub of agricultural sector occupying over 80% of its proportion of the sector’s output since 1988 (CBN, 2015). Some of these crops include: cassava, groundnut, tomato, cashew, maize, soybean, rice, oil palm, cotton, yam, and cocoa, among others. According to CBN (2017), the major six producing crops in Nigeria are: cassava, yam, maize, guinea corn, cotton and groundnut.
Economic growth in Nigeria has been insufficient to achieve a steady growth (United States Agency for International Development-USAID, 2016). Hence, several attempts and programs have been established in the quest to accelerate economic growth of Nigeria. Among them are: National Accelerated Food Production Programme (NAFPP) 1972-1973 and Operation Feed the Nation (OFN) 1976-1980 to make Nigeria self-sufficient in food production, Green Revolution Programme (GRP) 1981-1983 to curtail food importation through boosting crop production, and promoting big mechanized farming, and “Go Back to Land” Programme 1983-1985 for making farmers out of all Nigerians. Recent programmes include: National Economic Empowerment and Development Strategy (NEEDS) in 1999 and National Special Programme on Food Security (NSPFS) in 2002 and Root and Tuber Expansion Programme (RTEP) in 2003, among others. According to Okafor (2017), these have been affected by absence of involvement of all stakeholders, short duration of the policies, and inconsistency of regional agriculture development policies with the national policies and inadequate monitoring and evaluation of the programmes.
The paper is structured into five parts. After the introduction is literature review, followed by Methodology of the study, Data analysis and results, and conclusion.
2.1 Conceptual Review
Crop production is the science dealing with the cultivation of crops and vegetables on a field scale, either under rain-fed or irrigation conditions (Frazenburg & Lötter, 2018). These crops are mainly annuals cultivated for food and commercials. It is the cultivation of plants both on land and riverine areas. These are planted, tendered (weeding, fertilizer application, trimming etc) and harvested when due. Crops usually planted in Nigeria among others include: rubber, beans, rice, cocoa, citrus crops, guinea corn, maize, wheat, cassava, palm, yam, kola, tomatoes etc. Broadly, these crops are grouped into cereals, roots and tuber, vegetables oil, pulses and nuts, fruits and sugars, vegetables and spices and forest crops (Babalola, 2002).
Crop production is one of the components of agriculture. It is a branch of agriculture that deals with growing crops for use as food and fiber. It includes grains, cotton, tobacco, fruits, vegetables, nuts and plants. Different crops grow best in different areas of the country. Warmer climates are ideal for growing citrus crops; northern states are best for growing apples and blueberries and the Midwest is ideal for growing grains, including wheat (Agromix, 2018). According to him, crop producers usually work from sunrise to sunset during planting and harvesting seasons and they sell the crops produced, plan crops for the next season and repair machinery (Agromix, 2018).
Economic growth is the increase in market value of goods and services produced by an economy over time. It is usually measured as the percentage rate of increase in real Gross Domestic Product (GDP) or real GDP. It is the rate at which GDP is increasing –positive economic growth or decreasing –negative economic growth. Economic growth occur when there is a positive increase in an economic variable, real or nominal, normally persisting over successive periods (Esseins, 2001). Jhingan (2004) sees economic growth as an expansion in one or more areas without a change in its structure. Milton (1980) views economic growth as the rate of increase in an economy`s full employment, real output or income over time. According to Jhingan (2007) economic growth is related to a quantitative sustained increase in the countries per capita output or income accompanied by expansion in its labour force, consumption, capital and the volume of trade. Todaro (1981) sees economic growth as a steady process of increasing the productivity capacity of the economy and hence of increasing national income, Economic growth is related to high rate of growth of per capita output and high rate of increase in total factor productivity.
According to Guru (2016), economic growth has been defined in two ways. In one way, economic growth is defined as sustained annual increases in an economy’s real national income over a long period of time. In other words, economic growth means rising trend of net national product at constant prices. This definition has been criticized by some economists as inadequate and unsatisfactory. They argue that total national income may be increasing and yet the standard of living of the people may be falling. This can happen when the population is increasing at a faster rate than total national income. Hence, the second and better way of defining economic growth is to do so in terms of per capita income. According to the second view of Guru (2016), “economic growth means the annual increase in real per capita income of a country over the long period. Thus, Professor Arthur Lewis says that economic growth means the growth of output per head of population. Since the main aim of economic growth is to raise the standards of living of the people, therefore the second way of defining economic growth which runs in terms of per capita income or output is better (Guru, 2016). To him, the rates of economic growth are measured both in terms of increase in overall Gross National Product (GNP) or Net National Product (NNP) and increase in per capita income.
Crop Production and Economic Growth
Generally, there are different channels through which crop production affects the growth of an economy. This can be presented in Figure 1.
(See in PDF file)
Figure 1: Conceptual Framework of Crop Production and Economic Growth
Source: Author’s Conception, 2019
The crop production-economic growth linkage as illustrated in Figure 1, can best explain the effects of crop production to economic growth. Crop products can be categorized into food crops, cash crops and export crops. The improvement in cash crops and export crops is expected to improve income and growth. This improves the growth of the sector and add more value to agricultural products. Crop production also increases the household consumption of the food crops and proportion of it for commercial purpose (cash crops and export crops) which may boost foreign earnings and increase the level of income. Increases in households’ consumption and foreign earnings have the capacity of boosting economic growth (Karim, Karim & Zaidi, 2012).
2.2 Theoretical Review
The theories reviewed in this study include: unbalanced growth theory, and the Cobb-Douglas production function theory.
Scholars such as Hirschman (1956) and Rostow (1956) propounded the theory of unbalanced growth as a strategy of development to be used by the underdeveloped countries (Rostow, 1956; Singer, 1958; Hirschman, 1969; Streeten, 1969; Nath, 1971). This theory stresses the need for investment in strategic sectors of the economy instead of all the sectors simultaneously. According to this theory, the other sectors would automatically develop themselves through what is known as “linkages effect” (Saliminezhad & Lisaniler, 2017). The theory argues that creating imbalances in the system is the best strategy for growth owing to the lack of availability of resources in the less developed countries, the little that is available must be used efficiently. Accordingly, strategic sectors in the economy should get priority or precedence over others where income is concerned.Unbalanced growth theory became more appropriate strategy to initiate and accelerate economic growth. Countries such as Taiwan, Japan, and South Korea that followed Hirschman’s strategy have been the most successful in their development policies (Amores, 2012). In a similar fashion, this study intends to investigate the validity of the unbalanced growth theory proposed by Hirschman and identify the strategic sector(s) in Nigeria.
The unbalanced growth theory is considered applicable in this research because it supports investment in key sectors of the economy which when developed will influence and precipitate growth in other sectors through benefits occurring from the developed sector via forward and backward effects (Hirschman, 1958). It implies then that if the government can focus on agricultural sector and particularly crop production especially now that there is dwindling in the oil prices, it will be used to develop other sectors and this would lead to overall economic growth of the Nigerian economy.
The Cobb-Douglas production function is based on the empirical study of the American manufacturing industry made by Douglas and Cobb in 1928 (Tan, 2008). In 1928, Charles Cobb and Paul Douglas published in a study in which they modeled the growth of the American economy during the period 1899-1922 (Ioan & Ioan, 2015). It is a linear homogenous production function of degree one which takes into account two inputs, labour and capital for the entire output of the crop production (Moffatt, 2019). The Cobb-Douglas production function is expressed by Q = A Lα Cβ, where Q is output, L and C are inputs of labour and capital respectively. A, α and β are positive parameters where α > 0 and β>0. The equation tells that output depends directly on L and C, and that part of the output that cannot be explained by L and C is explained by A which is residual, often called technical change. The coefficient of labour α measures the percentages increase in Q that would result from a percent increase in L, while holding C as constant. Similarly β is the percentage increase in Q that would result from a percent increase in C while holding L as constant (Tan, 2008). The relevance of the theory is that it is used in analyses of economies of modern, developed, and stable nations around the world in terms of inputs and output models. This study therefore employs the Cobb-Douglas production function theory for the work.
2.3 Empirical Review
Sertoğlu, Ugural and Bekun (2017) examined the impact of agricultural sector on the economic growth of Nigeria using time series data from 1981 to 2013. The study used Vector Autoregressive (VAR) framework for the estimation. Findings from the study revealed that real gross domestic product; agricultural output and oil rents have a long-run equilibrium relationship. Vector error correction model result shows that the speed of adjustment of the variables towards their long run equilibrium path was low, though agricultural output had a positive impact on economic growth. The study considered the aggregates from agricultural sector and analysed its effect on economic growth. Even though, the study used VAR framework which is a system of equations, it failed to account for the transmission effect of agriculture to economic growth and the instantaneous response of agriculture to shocks in economic growth.
Ewetan, Fakile, Urhie and Oduntan (2017) examined the long run relationship between agricultural output and economic growth in Nigeria for the period 1981 to 2014 using time series data. The study used VAR framework and causality test. Results from Johansen maximum likelihood co-integration approach and Vector error correction model support evidence of long run relationship between agricultural output and economic growth in Nigeria. The result from granger causality test also confirms the co-integration results indicating the existence of causality between agricultural output and economic growth in Nigeria. The nature of the causality however depends on the variable used to measure agricultural output. This study failed to decompose the different activities under agricultural sector and excluded important variables like labour from their model. More so, the nature of the long-run relationship was not critically appraised and the transmission effect of agriculture to economic growth was not attempted.
Rahman (2017) examined the role of agriculture in Bangladesh economy and particularly uncovering the problems and challenges. The study used percentages, graphs and tables. The data covered 2007 to 2015. The study found that agriculture plays a key role in the overall economic performance of Bangladesh not only in terms of its contribution to GDP but also as a major source of foreign exchange earnings and in providing employment to a large segment of the population and particularly the poor. The study was however, qualitative in nature and lack quantitative approach of analyzing the impact of crop production on economic growth. Besides, the study examined the direct effects of agriculture to GDP, foreign earnings and employment without accounting for the indirect effects of agriculture to overall economic performance of Bangladesh.
Michael (2017) investigated the contribution of agricultural sector output to the growth of domestic economy in Nigeria for the period 1980 to 2014. The study used Cointegration test, Vector Error Correction Model (VECM) and Granger causality test were utilized in the analysis. The variables employed in the investigation include Real Gross Domestic Product (RGDP), value of Agricultural Output (VAO), Foreign Private Investment (FPI) and financial development (FD). A stationarity test was conducted through the application of the Augmented Dickey-Fuller (ADF) stationarity test, and the result showed that all the variables except RGDP were non-stationary at level; however, the variables such as VAO, FPI and FD became stationary after first differencing. The co-integration result indicated long run equilibrium relationship among the variables under study. The study also showed that value of agricultural output (VAO) has positive and insignificant contribution to real GDP. Furthermore, the Pairwise Granger causality result showed that significant causality exist between the two variables, with causality running from agricultural output to RGDP. It therefore, implies that agricultural sector output contributed positively and insignificantly to the growth of Nigerian domestic economy. The study was however, used pairwise granger causality without accounting for the lags as reported by the stationarity of the series preferably, Granger causality within VAR environment. The study also examined the direct effects of agriculture to GDP without accounting for the indirect effects of agriculture to economic growth in Nigeria.
Bekun (2015) examined the impact of agricultural sector on the economic growth of Nigeria. The study is conducted using annual time series data from 1981 to 2013. The study employs Johansen multivariate co-integration test and Vector Error Correction model (VECM) as the estimation techniques. The results of the study reveals that Real Gross Domestic Product (RGDP), agricultural output and oil rents have a long-run equilibrium relationship according to the Johansen Multivariate co-integration test whereas, the VECM result shows that the speed of adjustment of the variables towards their long-run equilibrium path was low. This study also failed to decompose the different activities under agricultural sector. More so, the transmission effect of agriculture to economic growth was not examined.
Chongela (2015) estimated the contribution of the agriculture sector to the Tanzanian economy. The study focused on time series data collected for the period of 1981 to 2010 in Tanzania Mainland. The study used the Mean Model to estimate the contribution of agriculture sector to the Tanzanian economy. Moreover, the significant contribution of crops, livestock and fisheries subsectors to the Agriculture Gross Domestic Product (AgGDP) was calibrated by a Multiple Regression Model. The study showed that agriculture sector is the key contributor to the national economy contributed by crops subsector, livestock subsector and fisheries subsector. This study made efforts with regards to decomposing the agricultural sector into: crops, livestock and fisheries however, the transmission effect or inter-temporal effects of crop production to economic growth was not examined.
Lyatuu, Nie and Fang (2015) assessed agriculture’s role during economic growth and its impact on poverty reduction. The study used OLS for the estimation covering 1965 to 2015. The study found that increase in population and poor public services in rural exacerbating poverty and accelerate shifting from agriculture to non-agriculture activities especially educated youth, thus, structural transformation. The study also found that agriculture contributes to the growth of the economy.
Oyakhilomen and Zibah (2014) examined the relationship between agricultural production and the growth of Nigerian economy with focus on poverty reduction. The study used time series data for the analysis. The study employed unit root test for stationarity and the bounds (ARDL) testing approach to cointegration. The result showed that agricultural production was significant in influencing the favourable trend of economic growth in Nigeria. The study recommended that pro poor policies should be designed for alleviating rural poverty through increased investments in agricultural development by the public and private sector. The study however did not disaggregate the impact of different categories of the agricultural production on the level of economic growth in Nigeria. The study also treated the model estimated as a single equation model and not two way effects in system of equations.
3. METHODOLOGY
Research Design
The study will use correlational research design. This research design is preferred because it is the most appropriate technique in dealing with examining the relationship between two or more variables. It is more appropriate to help the researcher in gauging results according to the objectives of this research.
Nature and Sources of Data
The study used basically secondary data. These data include: data for gross domestic product at current basic prices, food crops, cash crops, cassava output, cocoyam output, cotton output, groundnut output, guinea corn output, maize output, millet output, rice output, yam output, area harvested, the amount of rainfall, yield per unit of area and amount of fertilizer used were sourced from Central Bank of Nigeria Statistical Bulletin, crop pest interaction from Food and Agricultural Organisation of the United Nation Surveys and the level of carbon emission and agricultural labour force from the World Bank Development Indicators. The data were sourced from Central Bank of Nigeria Bulletin, Food and Agricultural Organisation of the United Nation and World Development Indicators site. The study therefore used internet and statistical bulletins for the data collection.
Model Specification
The model for the study is calibrated from the theory of unbalanced growth. Following the unbalanced growth theory, the growth of an economy is a function of growth in a particular sector of the economy. Adopting the specification by Ekine and Onu (2018) with modifications, the model can be expressed in a functional form as:
…………………………….. (1)
Where GDP (Gross Domestic Product) is a proxy for economic growth (GROWTH) and CROP is crop output in monetary terms.
However, decomposing or disaggregating the crop output into several crops which include: cash crops, and food crops. This can be specified as:
…………………… (2)
Where cash is crops, and is food crops. Thus, taking natural logarithm and specifying the stochastic form of equation (2), it can be restated as:
… (3)
Where is the intercept, is the parameters to be estimated, is natural logarithm and is the stochastic error term.
The study also captures the impact of individual crops on economic growth. Hence, the component of crop production in equation (1) is disaggregated into the major crops produced in Nigeria that has at least average output capacity margin between 2010 and 2018. The major crops include: Cassava, Cocoyam, Cotton, Groundnut, Guinea Corn, Maize, Millet, Rice and Yam. The definitional form of the model can be captured as:
……………(4)
Where CAS is cassava output, CCY is cocoyam output, CTN is cotton output, GNC is the Guinea corn output, GNT is groundnut, MAZ is maize output, MLT is millet output, RCE is rice output and YAM is yam output. Thus, taking natural logarithm and specifying the stochastic form of equation (4), it can be restated as:
……. (5)
Where is the intercept, is the parameters to be estimated, is natural logarithm and is the stochastic error term.
Methods of Data Analysis
The study employed both descriptive statistics and the econometric tools in analyzing the data. The descriptive tools summarizes the basic statistical features of the data under consideration including the mean, the minimum and maximum values, standard deviation, skewness, kurtosis and the Jarque-Bera test for the data. These descriptive statistics provide a historical background for the behavior of our data. While the econometric tools that were used include Unit Root test and Vector Autoregressive Models. The unit root tests considered include the conventional unit root tests; namely Augmented Dickey-Fuller (ADF) and Ng-Perron (Ng-P) unit root tests. They were used to test for the stationarity of the data.
4. DATA ANALYSIS AND RESULTS
The data used for the analysis include data on Gross Domestic Product at current basic prices (GDP), Crop Production (CROP), Cash Crops (CCP), Food Crops (FCP), Cassava Output (CAS), Cocoyam Output (CCY), Cotton Output (CTN), Groundnut Output (GNT), Guinea corn Output (GNC), Maize Output (MAZ), Millet Output (MLT), Rice Output (RCE), Yam Output (YAM).
Data on gross domestic product at current basic prices, crop production, cash crops, food crops, cassava output, cocoyam output, cotton output, groundnut output, guinea corn output, maize output, millet output, rice output, yam output were sourced from the Central Bank.
Descriptive Statistics
The summary results of descriptive statistics of the variables are presented in Table 1.
Table 1: Descriptive Statistics
CAS | CCY | CTN | GNC | GNT | MAZ | MLT | RCE | YAM | |
Mean | 2074.74 | 160.91 | 300.48 | 307.19 | 179.67 | 419.86 | 264.82 | 191.64 | 1372.62 |
Median | 229.61 | 74.18 | 123.77 | 93.9 | 86.29 | 149.51 | 109.02 | 78.22 | 262.96 |
Maximum | 7771.58 | 630.89 | 1178.29 | 1264.19 | 680.13 | 1707.69 | 1037.53 | 755.39 | 6059.17 |
Minimum | 1.3 | 1.3 | 1.41 | 1.47 | 0.94 | 1.29 | 2.15 | 1 | 1.49 |
Std. Dev. | 2632.5 | 188.07 | 356.29 | 385.63 | 203.99 | 513.26 | 313.27 | 227.15 | 1869.48 |
Skewness | 0.84 | 1.15 | 1.13 | 1.2 | 1.08 | 1.16 | 1.13 | 1.13 | 1.24 |
Kurtosis | 2.11 | 3.11 | 3.04 | 3.13 | 2.99 | 3.1 | 3.05 | 3.06 | 3.18 |
Jarque-Bera | 5.75 | 8.36 | 8.05 | 9.11 | 7.4 | 8.51 | 8.14 | 8.1 | 9.85 |
Probability | 0.06 | 0.02 | 0.02 | 0.01 | 0.02 | 0.01 | 0.02 | 0.02 | 0.01 |
Sum | 78840.26 | 6114.54 | 11418.14 | 11673.18 | 6827.33 | 15954.56 | 10063 | 7282.38 | 52159.73 |
Sum Sq. Dev. |
2.56E+08 |
1308732 |
4696985 |
5502180 |
1539570 |
9747266 |
3631115 |
1909066 |
1.29E+08 |
Observations | 38 | 38 | 38 | 38 | 38 | 38 | 38 | 38 | 38 |
The result of the descriptive statistics in Table 1 reveals that cassava output (CAS), cocoyam output (CCY), cotton output (CTN), guinea corn output (GNC), groundnut output (GNT), and maize output (MAZ) averaged ₦2,074.74 billion, ₦160.91 billion, ₦300.48 billion, ₦307.19 billion, ₦179.67 billion and ₦419.86 billion while millet output (MLT), rice output (RCE) and yam output (YAM) averaged ₦264.82 billion, ₦191.64 billion and ₦1,372.62 billion respectively. From the average output of crops, cassava and yam output are among the top crops produced in Nigeria within the study period. All the crops had minimum values in 1981 while the maximum values were recorded in 2018 except for cassava output that had its maximum value in 2000. This implies that the series trended upward.
Gross domestic product recorded its maximum value in 2018 with its minimum value in 1981. The study also found that all the individual crops (cash crops, food crops, cassava output, cocoyam output, cotton output, groundnut output, guinea corn output, maize output, millet output, rice output, and yam output) are positively skewed. More so, gross domestic product at current basic prices, crop production, are positively skewed. This implies that the data distribution for the series have the tail on the right side of the distribution that are longer or fatter. This means that the averages and median values are greater than the values with highest frequency which simply show that the data distribution are titled towards large values.
The Jarque-Berra statistics for all individual crops (cassava output, cocoyam output, cotton output, groundnut output, guinea corn output, maize output, millet output, rice output, yam output) show that their probability value less greater than 0.05, indicating that, the data distribution are not normally distributed. More so, the Jargue-Berra statistics for crop production, gross domestic product are with probability values from Jargue-Berra statistics that are less than 0.05 implying that the distributions can be considered not normal.
Correlation Test Analysis
Table 2: Correlation Test Results
lnGDP | lnCAS | lnCCY | LnCTN | lnGNT | lnGNC | lnMAZ | lnMLT | lnRCE | lnYAM | |
lnGDP | 1 | |||||||||
lnCAS | 0.55 | 1 | ||||||||
lnCCY | 0.59 | 0.59 | 1 | |||||||
lnCTN | 0.59 | 0.59 | 0.56 | 1 | ||||||
lnGNT | 0.48 | 0.49 | 0.45 | 0.56 | 1 | |||||
lnGNC | 0.55 | 0.44 | 0.45 | 0.45 | 0.67 | 1 | ||||
lnMAZ | 0.69 | 0.55 | 0.56 | 0.44 | 0.44 | 0.67 | 1 | |||
lnMLT | 0.66 | 0.55 | 0.56 | 0.44 | 0.45 | 0.56 | 0.56 | 1 | ||
lnRCE | 0.59 | 0.44 | 0.44 | 0.67 | 0.67 | 0.67 | 0.56 | 0.56 | 1 | |
lnYAM | 0.56 | 0.45 | 0.44 | 0.56 | 0.55 | 0.56 | 0.56 | 0.56 | 0.56 | 1 |
Source: Extraction from E-views 10 Output
The result in Table 2 reveals that the correlation coefficients among the variables are not high that could result to multicollinearity among the variables for the study. This is because there is moderate correlation between most of the variables except for few variables. However, these are not capable of creating the problem of single equation matrix or resulting to impossibility of determining estimates from the model.
Unit Root Test Result
The result obtained from the Augmented Dickey-Fuller unit root test for all the time series used in the estimation are presented in the Table 3.
Table 3: ADF Unit Root Test Results
Variables | ADF Statistic at Level | ADF Statistic at First Difference | 1% level | 5% level | 10%
level |
Prob. |
lnCAS | -1.70494 | -3.43438 | -3.62678 | -2.94584 | -2.61153 | 0.0161** |
lnCCY | -1.63546 | -4.24065 | -3.62678 | -2.94584 | -2.61153 | 0.0020*** |
lnCROP | -1.90072 | -4.13343 | -3.62678 | -2.94584 | -2.61153 | 0.0027*** |
lnCTN | -2.16729 | -4.26071 | -3.62678 | -2.94584 | -2.61153 | 0.0019*** |
lnGDP | -1.15519 | -3.18046 | -3.62678 | -2.94584 | -2.61153 | 0.0295** |
lnGNC | -1.95348 | -4.61274 | -3.62678 | -2.94584 | -2.61153 | 0.0007*** |
lnGNT | -2.47394 | -4.20881 | -3.62678 | -2.94584 | -2.61153 | 0.0022*** |
lnMAZ | -2.33981 | -4.20304 | -3.62678 | -2.94584 | -2.61153 | 0.0022*** |
lnMLT | -1.60820 | -4.47271 | -3.62678 | -2.94584 | -2.61153 | 0.0010*** |
lnRCE | -2.06682 | -4.31258 | -3.62678 | -2.94584 | -2.61153 | 0.0016*** |
lnYAM | -2.13211 | -4.42686 | -3.62678 | -2.94584 | -2.61153 | 0.0012*** |
Source: Extraction from E-views 10 Output
From the results of the ADF unit root in Table 6, all the variables are integrated at the first difference that is, I (1). This is because the probability value of the series are less than their 0.05 critical values respectively at first difference. However, none of the series were stationary at level at 5% level of significance and that necessitated the differencing of the series. Given that all the series are integrated at first difference.
Cointegration Analysis
The result in Table 4 reveals that there is co-integration among the variables. The result reveals ten co-integrating equations from both Trace statistics and Max-Eigen statistics.
Table 4: Co-integration Test Results
Hypo- thesized No. of
CE(s) |
Eigen Value | Trace Statistic | 0.05
Critical Value |
Prob.** | Hypo- thesized No. of
CE(s) |
Eigen value | Max- Eigen Statistic | 0.05
Critical Value |
Prob.** |
None * | 0.984830 | 635.4209 | 239.2354 | 0.0000 | None * | 0.984830 | 150.7840 | 64.50472 | 0.0000 |
At most 1 * | 0.941588 | 484.6369 | 197.3709 | 0.0001 | At most 1 * | 0.941588 | 102.2484 | 58.43354 | 0.0000 |
At most 2 * | 0.936671 | 382.3885 | 159.5297 | 0.0000 | At most 2 * | 0.936671 | 99.33860 | 52.36261 | 0.0000 |
At most 3 * | 0.849925 | 283.0499 | 125.6154 | 0.0000 | At most 3 * | 0.849925 | 68.27827 | 46.23142 | 0.0001 |
At most 4 * | 0.809502 | 214.7716 | 95.75366 | 0.0000 | At most 4 * | 0.809502 | 59.69215 | 40.07757 | 0.0001 |
At most 5 * | 0.805870 | 155.0795 | 69.81889 | 0.0000 | At most 5 * | 0.805870 | 59.01224 | 33.87687 | 0.0000 |
At most 6 * | 0.713114 | 96.06724 | 47.85613 | 0.0000 | At most 6 * | 0.713114 | 44.95214 | 27.58434 | 0.0001 |
At most 7 * | 0.525742 | 51.11510 | 29.79707 | 0.0001 | At most 7 * | 0.525742 | 26.85616 | 21.13162 | 0.0070 |
At most 8 * | 0.430289 | 24.25895 | 15.49471 | 0.0019 | At most 8 * | 0.430289 | 20.25451 | 14.26460 | 0.0050 |
At most 9 * | 0.105271 | 4.004436 | 3.841466 | 0.0454 | At most 9 * | 0.105271 | 4.004436 | 3.841466 | 0.0454 |
Source: E-views Results Output, 2020
The result reveals ten co-integrating equations from both Trace statistics and Max-Eigen statistics. This is because the revealed trace statistics and Max-Eigen statistics are greater than the 5% critical value at the respective hypothesized number of co-integrating equations. Therefore, the study infers that there is evidence of co-integration among the variables from the model. This implies that r=there is long-run relationship between crop production and economic growth in Nigeria.
VAR Lag Order Selection Criteria
The results of the VAR lag selection criteria are presented in Table 5. The VAR lag selection criterion test determines the optimal lag that would yield robust results.
Table 5: VAR Lag Order Selection Results
Lag | LogL | LR | FPE | AIC | SC | HQ |
0 | 560.2136 | NA | 2.51e-26 | -30.5674 | -30.1276 | -30.4139 |
1 | 1158.058 | 830.3393 | 2.99e-38 | -64.88593* | -55.64874* | -61.66190* |
2 | 1377.947 | 183.2407* | 1.69e-40* | -58.2254 | -53.38691 | -56.5367 |
Source: E-views Result Output 2020
* indicates lag order selected by the criterion. LR: sequential modified LR test statistic (each test at 5% level), FPE: Final prediction error, AIC: Akaike information criterion, SC: Schwarz information criterion and HQ: Hannan-Quinn information criterion.
The result presented in Table 5 show that lag one (1) is the optimal lag for the model because it has the least AIC, SC and HQ relative to the other lags. Based on the selected criteria (AIC and SC) for this study.
Impact of Crop Production on Economic Growth in Nigeria
In order to determine the long run impact of crop production on economic growth in Nigeria, the study used the long-run estimates from Vector Error Correction Model (VECM) given that the series were co-integrated. The equations for the long-run impact of crop production on economic growth in Nigeria is stated as:
Note: standard errors are in parenthesis () while t-statistics are in brackets [].
From the long-run estimates, in determining the impact of crop production on economic growth in Nigeria, the estimated coefficients of cassava output, cocoyam output, cotton output, groundnut output, guinea corn output, maize output, millet output, rice output and yam output are all positive. This implies that the estimates are theoretically plausible in the long-run. The estimates further show that apart from cotton output, all other crops are statistically significant at influencing the growth of the Nigerian economy at 5% level of significance. This means that increase in crops production exert strong positive influence on economic growth in Nigeria in the long-run and vice versa, ceteris paribus. The implication is that crop production has strong positive influence on the performance of the Nigerian economy. This finding is consistent with Ewetan, Fakile, Urhie and Oduntan (2017) and Okoro (2011), who found positive relationship between crop productions and output in Nigeria in the long-run. The weak influence of cotton on economic growth may be attributed to the lack of takeoff from producers as a result of the nature of textile industry, hence, it has the capacity of discouraging local farmers.
The result of the vector error-correction test also measures the short-run relationship amongst variables. The coefficient of error-correction variable gives the percentage of the discrepancy between the variables that can be eliminated in the next time period. The results are summarized in Table 6
Table 6: Model Equation One: Short-run VAR Estimates
Variables | Coefficient | Std. Error | t-Statistic | Prob. |
CointEq1 | -0.00791 | 0.002756 | -2.86879 | 0.0045*** |
D(LNGDP(-1)) | 0.198463 | 0.296773 | 0.668737 | 0.5043 |
D(LNCAS(-1)) | 0.00077 | 0.168684 | 0.00455 | 0.9964 |
D(LNCCY(-1)) | 0.319909 | 0.952707 | 0.33579 | 0.7373 |
D(LNCTN(-1)) | -7.39101 | 6.400325 | -1.15479 | 0.2493 |
D(LNGNC(-1)) | 1.79765 | 1.531107 | 1.17408 | 0.2415 |
D(LNGNT(-1)) | 3.186203 | 1.346856 | 2.36566 | 0.0188** |
D(LNMAZ(-1)) | 6.14971 | 3.998413 | 1.53804 | 0.1254 |
D(LNMLT(-1)) | 3.45527 | 2.198927 | 1.57135 | 0.1174 |
D(LNRCE(-1)) | 14.47205 | 10.86362 | 1.332157 | 0.1841 |
D(LNYAM(-1)) | 0.798687 | 0.534112 | 1.495356 | 0.1361 |
C | 0.18144 | 0.051903 | 3.495757 | 0.0006*** |
Source: E-views Results Output, 2020
The asterisk (*, **, ***) denotes rejection of the unit root hypothesis at 10%, 5% and 1% critical levels respectively.
The short run estimates in Table 4.6 show that cassava output, cocoyam output, groundnut output, guinea corn output, maize output, millet output, rice output and yam output are theoretically plausible in the short-run while the estimated coefficient for cotton output is not theoretically plausible. From the estimates, only groundnut output that is statistically significant at 5% level of significance in the short-run. This implies that increase in groundnut production has strong positive influence on the performance of the Nigerian economy in the short-run and vice versa, ceteris paribus.The estimated coefficient of error correction term from model equation one is negative (- 0.007907) and statistically significant at 5% level of significance. This indicates that in case of any deviation, the long run equilibrium may converge at the speed of 0.79%each period (that is each year). This shows that the speed of adjustment towards long-run equilibrium in economic growth in Nigeria even when there is initial disequilibrium is slowly at the rate of 0.79%. It is also glaring from the coefficient of multiple determinations (R2) has a good fit as the independent variables were found to jointly explain 58.75% of the movement in the dependent variable with the adjusted R-squared of 49.84%. The overall significance of the models is explained by the F-statistic of 3.107199.
Table 7: Short-run Impact of Crop Production on Economic Growth in Nigeria
Variables | Coefficient | Std. Error | t-Statistic | Prob. |
CointEq1 | -0.00791 | 0.002756 | -2.86879 | 0.0045*** |
D(LNGDP(-1)) | 0.198463 | 0.296773 | 0.668737 | 0.5043 |
D(LNCAS(-1)) | 0.00077 | 0.168684 | 0.00455 | 0.9964 |
D(LNCCY(-1)) | 0.319909 | 0.952707 | 0.33579 | 0.7373 |
D(LNCTN(-1)) | -7.39101 | 6.400325 | -1.15479 | 0.2493 |
D(LNGNC(-1)) | 1.79765 | 1.531107 | 1.17408 | 0.2415 |
D(LNGNT(-1)) | 3.186203 | 1.346856 | 2.36566 | 0.0188** |
D(LNMAZ(-1)) | 6.14971 | 3.998413 | 1.53804 | 0.1254 |
D(LNMLT(-1)) | 3.45527 | 2.198927 | 1.57135 | 0.1174 |
D(LNRCE(-1)) | 14.47205 | 10.86362 | 1.332157 | 0.1841 |
D(LNYAM(-1)) | 0.798687 | 0.534112 | 1.495356 | 0.1361 |
C | 0.18144 | 0.051903 | 3.495757 | 0.0006*** |
Source: Extraction from E-views 10 Output
The asterisk (*, **, ***) denotes rejection of the unit root hypothesis at 10%, 5% and 1% critical levels respectively.
The short run estimates in Table 7 show that cassava output, cocoyam output, groundnut output, guinea corn output, maize output, millet output, rice output and yam output are theoretically plausible in the short-run while the estimated coefficient for cotton output is not theoretically plausible. From the estimates, only groundnut output that is statistically significant at 5% level of significance in the short-run. This implies that increase in groundnut production has strong positive influence on the performance of the Nigerian economy in the short-run and vice versa, ceteris paribus.
The estimated coefficient of error correction term is negative (-0.007907) and statistically significant at 5% level of significance. This indicates that in case of any deviation, the long run equilibrium may converge at the speed of 0.79% each period (that is each year). This shows that the speed of adjustment towards long-run equilibrium in economic growth in Nigeria even when there is initial disequilibrium is slowly at the rate of 0.79%. It is also glaring from the coefficient of multiple determinations (R2) has a good fit as the independent variables were found to jointly explain 58.75% of the movement in the dependent variable with the adjusted R-squared of 49.84%. The overall significance is explained by the F-statistic of 3.107199.
Impulse Responses Analysis of Economic Growth to Shocks in Crop Production
Figure 2: Impulse response of economic growth to shocks in crop production in Nigeria
Source: E-Views Result Output, 2020
From the impulse response of economic growth to shocks in crop production in Nigeria in a 10-year forecast period, the result shows that economic growth would response positively and permanently to own shock (figure 2). The study also found that economic growth would respond negatively to shock in production of cotton, maize, and rice in Nigeria but economic growth would respond positively to shock in the production of groundnut, yam, millet, and guinea corn. Shocks in millet exerts a temporary and initial negative effect on economic growth but reverts positive in the long-run. Shocks in cocoyam and cassava would have initial positive influence on the level of economic growth but turns slightly negative in the long-run.
Variance Decomposition Analysis
The results of the accumulated forecast error variance of economic growth to shocks in crop production in Nigeria are presented in Table 8 below.
Table 8: Accumulated Forecast Error Variance of Economic Growth to Shocks in Crop Production
Variance Decomposition of lnGDP: | |||||||||||
Period | lnGDP | lnCAS | lnCCY | lnCTN | lnGNC | lnGNT | lnMAZ | ||||
Shor-term | 84.83270 | 0.033408 | 0.067982 | 9.641819 | 2.935547 | 0.132501 | 0.498820 | ||||
Middle-term | 67.20251 | 0.212515 | 0.272889 | 22.90178 | 1.250281 | 0.079027 | 3.097321 | ||||
Long-term | 60.42985 | 0.349880 | 0.187900 | 25.60045 | 0.619974 | 0.506633 | 2.219937 | ||||
lnMLT | lnRCE | lnYAM | |||||||||
Shor-term | 1.112362 | 0.022595 | 0.722264 | ||||||||
Middle-term | 1.082008 | 2.099552 | 1.802116 | ||||||||
Long-term | 3.550649 | 5.465214 | 1.069512 | ||||||||
Analysis of the accumulated forecast error variance of economic growth in the medium-term period and 25.6% and 5.46% of the accumulated forecast error variance of economic growth in the long-term period respectively. This implies that variance in economic growth due to innovations in cotton production and rice production would increase overtime, however, cotton production would exert relatively higher variations in economic growth than the rice production. In terms of own shocks, the accumulated forecast error variance of economic growth to own shocks would account for 84.83% in the short-term, 67.2% in the medium-term and 60.42% in the long-term period respectively. This implies that variance in economic growth would decline over time to own shock. The result further shows that majority of the variations in economic growth would be accounted by own shocks throughout the forecast period.
Shocks in other variables would exert little variations in economic growth in Nigeria. For instance, the shocks in cassava, groundnut, and millet would exert increasing variations in economic growth while shocks in cocoa-yam, guinea-corn, maize, and yam would exert increasing variations in economic growth during the forecast period. However, these account for less than 5% variation in economic growth in Nigeria throughout the forecast period.
4.3 Discussion of Results and Findings
In examining the impact of crop production on economic growth in Nigeria, the study found that cassava output, cocoyam output, groundnut output, guinea corn output, maize output, millet output, rice output and yam output are theoretically plausible and statistically significant in the long-run at 5% level of significance while only cotton output is theoretically plausible in the long-run but not statistically significant in the long-run at 5% level of significance. This is in variance with the findings from Uma, Eboh and Obidike (2013), and Ekine and Onu (2018) who found that crop production does not have significant impact on economic growth in Nigeria. The studies however, aggregated the crop production without considering the individual crops impact on economic growth in Nigeria like in this study. The implication is that increase in the production of cassava, cocoyam, groundnut, guinea corn, maize, millet, rice and yam spurs economic growth in Nigeria in the long-run.
The study also found that groundnut contributes positively and significantly to the output of the Nigerian economy in the short-run at 5% level of significance. Only cotton that contributed negatively to output in Nigeria within the period under study due to the neglect of the activities and the nature of the textile industry in the country. All other individual crops are theoretically plausible but not statistically significant at influencing economic growth of the Nigerian economy. Previous studies have failed to account for the short-run and long-run impact of this crop production on economic growth in Nigeria. However, given that most used ordinary least squares, the estimates analyze only short-run relationship. Hence, the insignificant impact of most of the individual crops on economic growth in the short-run is consistent with the findings of Uma, Eboh and Obidike (2013), and Ekine and Onu (2018) who used ordinary least squares and found that crop production does not have significant impact on economic growth in Nigeria.
The study also assessed the residuals to establish whether the model is valid. The study, therefore, examined whether their estimates are reliable, normal and whether estimates can yield robust statistical inferences using their residuals. The study found that there is no evidence of serial correlation based on the results of the VEC serial correlation LM test for the models. The study also found that Vector Error Correction VEC residual normality and heteroscedasticity tests indicate absence of heteroscedasticity and that the residuals are normally distributed. The model is also proven dynamically stable by the results of the inverse roots of AR characteristic polynomial implying that the estimates produced by the model is reliable and can stand statistical inferences.
5. CONCLUSION AND RECOMMENDATIONS
The study concludes that crop production is very crucial to the growth of the Nigerian economy, and contributes greatly to the economic growth of the nation.
Given that crop production is very beneficial to the growth in Nigerian economy, the study recommends that the Nigerian government should encourage farmers in the production of crops. This can be done through supply of fertilizer to farmers since increase in fertilizer application enhances crop output in the country. This is because fertilizers increase farmers‟ productivity and helps increase production and improve food security. Farmers should to engage into large scale farming through Agric loans and grants. Also farmers should be educated on the use of improved seedlings and modern farming techniques.
Government should look into the insecurity ravishing the rural areas, hence discouraging farming. Also farmer/herders problem should be dealt with and resolved.
REFERENCES
- Abdulrahaman, A. A. & Kolawole, O. M. (2006). Traditional preparations and uses of maize in Nigeria. Ethnobotanical Leaflets, 10(1): 219-227.
- Adelman I. (1984). Beyond exported growth. World Development, 12(1), 937-949.
- Aditya, T (2019). Agricultural production and economic growth in Nigeria: Problem and prospect. Journal of International Agriculture, 3(3): 7-10.
- Agricultural Transformation Agenda (2011). We will grow Nigeria‟s Agricultural Agricultural transformation Blueprint. Abuja: Federal Ministry of Agriculture and Rural development.
- Agriculture Nigeria (2019). Crop Production. New York: Longman. Agromix (2018). Crop Production. Ibadan: University of Ibadan Press
- Amone, W. (2014). Agricultural Productivity and Economic Development in Uganda: An Inclusive Growth Analysis. Ibadan: University of Ibadan Press
- Anriquez G. & Stamoulis, K. (2007). Rural development and poverty reduction: is agriculture still the key?New York: Mc Craw Hill Companies
- Arokoyo, T. (2012). Challenges of Integrated Small Scale Farmers into the Agricultural Value Chain in Nigeria. Ibadan: University of Ibadan Press.
- Awokuse, T. O. (2008). Does Agriculture Really Matter for Economic Growth in Developing Countries?London: Macmillan Press.
- Ayoola, G. B. (2001). Essays on the agricultural economy. A Book ofReadings on Agricultural Development Policy andAdministration in Nigeria, Ibadan: TMA Publishers.
- Babalola, O. (2002). Nigeria Agriculture: Basis for Hope, Hurdles Against Hope for Tomorrow. University of Ibadan, Ibadan, Nigeria..
- Barro, R. J. (1991). Economic growth in a cross section of countries. Quarterly Journals of Economics, 106(2), 407-443.
- Bekun, F. V. (2015). The Contribution of Agricultural Sector on the Economic Growth of Nigeria (Masters Dissertation). Institute of Graduate Studies and Research.
- Byerlee, D., Janvry, A. & Sadoulet, E. (2008). Agriculture for Development: Toward a New Paradigm. Annual Review of Resource Economics, 1(1), 1-19.
- CBN (2012). Annual report and statement of Account for the year ended 31st Dec 2012.
- Retrieved from http://www.cbn.org.ng on August 3, 2019
- CBN (2016). Annual report and statement of Account for the year ended 31st Dec 2016.
- Retrieved from http://www.cbn.org.ng on May 16, 2019
- CBN (2017). Annual report and statement of Account for the year ended 31st Dec 2017.
- Retrieved from http://www.cbn.org.ng on May 16, 2019
- CBN-Central bank of Nigeria (2018). Annual report and statement of account for the year ended 31st2018. Retrieved from http://www.cbn.org.ngon May 16, 2019
- Central Bank of Nigeria (2015). CBN Statistical Bulletin. Abuja
- Cervantes-Godoy, D. & Dewbre, J. (2010). Economic Importance of Agriculturefor Poverty Reduction. USA: OECD Publication.
- Chongela, J. (2015). Contribution of agriculture sector to the Tanzanian economy.
- American Journal of Research Communication, 3(7), 57-70.
- Chukwu D, & Uju E. (2013). Does agriculture matter for economic development? Empirical evidence from Nigeria. Journal of Economics and Finance. 1(1), 61- 77.
- Courage, S. O. (2017). 11 Top Crops Farming and production in Nigeria; how to start & make money. Business Classroom for Africans (Since 2007). Retrieved from
https://www.africabusinessclassroom.com/crops-farming-production-nigeria- start-make-money/ on 22 April, 2019
- Degrande, , & Duguma, B., (2000). Adoption Potential of Rotational Hedge Row.
- Intercropping in Humid and Extension Network, 103(1), 1-7
- Diao, X. (2010). Economic Importance of Agriculture for Sustainable Development and Poverty Reduction: Findings from a Case Study of Ghana, Global Forum on Agriculture 29-30 November 2010 Policies for Agricultural Development, Poverty Reduction and Food Security OECD Headquarters, Paris.
- Dim, C. & Ezenekwe, U. (2013). Does Agriculture Matter for Economic Development? Empirical Evidence from Nigeria. Journal of Finance & Economics, 1(1), 61-77.
- Ekine, D. I. & Onu, C. (2018). The impact of agricultural output on economic growth in Nigeria (1981-2015). IOSR Journal of Economics and Finance, 4(1), 10-14
- El-Gizawy, N. (2016). Agronomy III (Crop Production). New York: Mc Craw Hill Companies.
- Enu, P. (2014). Analysis of the agricultural sector of Ghana and its economic impact on economic growth. Academic Research International, 5(4), 267-277
- Ewetan O., Fakile, N., Urhie, E. & Oduntan E. (2017). Agricultural output and economic growth in Nigeria. Journal of African Research in Business & Technology, 3(1), 1-11.
- FAO (2019a). Ghana at a glance. Food and Agriculture Organisation of the United Nations. Retrieved from http://www.fao.org/ghana/fao-in-ghana/ghana-at-a- glance/en/ on September 20, 2018.
- FAO (2019b). Programmes and Projects. Food and Agriculture Organisation of the United Nations. Retrieved from http://www.fao.org/nigeria/programmes-and- projects/programmes/en/on September 20,
- FAOSTAT (2013). A database of the Food and Agriculture Organization of the United Nations (FAO). Retrieved from http://faostat.fao.orgon September 20, 2018.
- Fleming, M. (1958). External economics and the doctrines of balance growth. The Economic Journal,24(1), 1-14.
- Food and Agricultural Organisation-FAO (2018). Countries by commodity, Food and Agricultural Organisation of the United Nations. UK: FAO Departments and Offices.
- Friedman M. (1980). Memorandum: Response to Questionnaire on monetary policy in treasury and civil service committee memoranda on monetary policy. Journal of Development Economics, 8(3), 55-61.
- Garvelink, .J. Wedding, K. & Hanson, S. (2012). Smallholder Agriculture: A Critical Factor in Poverty Reduction and Food Security in Africa. Washington, D.C.: Center for Strategic and International Studies.
- Gerald, G. (2000). Application to economics: Leontief Model. Groningen: University of Groningen Press.
- Greyling, J.C. (2012). The Role of the Agricultural Sector in the South African Economy (A Thesis). Department of Agricultural Economics, University of Stellenbosch.
- Gujarati, D.N & Porter, D.C. (2009). Basic Econometrics (5th). New York: Mc Craw Hill Companies.
- Hanjra, M.A. & Culas, R.J. (2011). The political economy of maize production and poverty reduction in Zambia. Analysis of the last 50 years. Journals of Asian and African Studies, 46(6), 546-566.
Impact of Crop Production on Economic Growth in Nigeria, 1981 – 2018
Joseph M. IBBIH1, Jorji Akudo NWOGU2
1Department of Economics, Faculty of Social Sciences, Nassarawa State University, Keffi Nigeria
2Department of Economics, Federal College of Education (Technical) Gusau, Zamfara State, Nigeria
Vol 4 No 5 (2024): Volume 04 Issue 05 May 2024
Article Date Published : 10 May 2024 | Page No.: 348-360
Abstract :
This study examined the impact of crop production on economic growth in Nigeria utilizing annual time series data from 1981 to 2018. The study used Johansen cointegration test, Vector Error Correction test, the impulse response functions and variance decomposition for data analysis after the data series were checked against unit root problem using the Augmented Dickey Fuller unit root test. The study tested and found that increase in the production of cassava, cocoyam, groundnut, guinea corn, maize, millet, rice and yam spurs economic growth in Nigeria in the long-run except for cotton production and with only groundnut production contributing significantly to the output of the Nigerian economy in the short- run. The study, therefore recommends that the Nigerian government should encourage farmers in the production of crops through supply of fertilizer to increase their productivity and food output while improving their use of modern seedlings and technology in the production that may in turn increase the crop production while farmers should be encouraged to adapt best practices in farming to maximize crop yield.
Keywords :
References :
- Abdulrahaman, A. A. & Kolawole, O. M. (2006). Traditional preparations and uses of maize in Nigeria. Ethnobotanical Leaflets, 10(1): 219-227.
- Adelman I. (1984). Beyond exported growth. World Development, 12(1), 937-949.
- Aditya, T (2019). Agricultural production and economic growth in Nigeria: Problem and prospect. Journal of International Agriculture, 3(3): 7-10.
- Agricultural Transformation Agenda (2011). We will grow Nigeria‟s Agricultural Agricultural transformation Blueprint. Abuja: Federal Ministry of Agriculture and Rural development.
- Agriculture Nigeria (2019). Crop Production. New York: Longman. Agromix (2018). Crop Production. Ibadan: University of Ibadan Press
- Amone, W. (2014). Agricultural Productivity and Economic Development in Uganda: An Inclusive Growth Analysis. Ibadan: University of Ibadan Press
- Anriquez G. & Stamoulis, K. (2007). Rural development and poverty reduction: is agriculture still the key?New York: Mc Craw Hill Companies
- Arokoyo, T. (2012). Challenges of Integrated Small Scale Farmers into the Agricultural Value Chain in Nigeria. Ibadan: University of Ibadan Press.
- Awokuse, T. O. (2008). Does Agriculture Really Matter for Economic Growth in Developing Countries?London: Macmillan Press.
- Ayoola, G. B. (2001). Essays on the agricultural economy. A Book ofReadings on Agricultural Development Policy andAdministration in Nigeria, Ibadan: TMA Publishers.
- Babalola, O. (2002). Nigeria Agriculture: Basis for Hope, Hurdles Against Hope for Tomorrow. University of Ibadan, Ibadan, Nigeria..
- Barro, R. J. (1991). Economic growth in a cross section of countries. Quarterly Journals of Economics, 106(2), 407-443.
- Bekun, F. V. (2015). The Contribution of Agricultural Sector on the Economic Growth of Nigeria (Masters Dissertation). Institute of Graduate Studies and Research.
- Byerlee, D., Janvry, A. & Sadoulet, E. (2008). Agriculture for Development: Toward a New Paradigm. Annual Review of Resource Economics, 1(1), 1-19.
- CBN (2012). Annual report and statement of Account for the year ended 31st Dec 2012.
- Retrieved from http://www.cbn.org.ng on August 3, 2019
- CBN (2016). Annual report and statement of Account for the year ended 31st Dec 2016.
- Retrieved from http://www.cbn.org.ng on May 16, 2019
- CBN (2017). Annual report and statement of Account for the year ended 31st Dec 2017.
- Retrieved from http://www.cbn.org.ng on May 16, 2019
- CBN-Central bank of Nigeria (2018). Annual report and statement of account for the year ended 31st2018. Retrieved from http://www.cbn.org.ngon May 16, 2019
- Central Bank of Nigeria (2015). CBN Statistical Bulletin. Abuja
- Cervantes-Godoy, D. & Dewbre, J. (2010). Economic Importance of Agriculturefor Poverty Reduction. USA: OECD Publication.
- Chongela, J. (2015). Contribution of agriculture sector to the Tanzanian economy.
- American Journal of Research Communication, 3(7), 57-70.
- Chukwu D, & Uju E. (2013). Does agriculture matter for economic development? Empirical evidence from Nigeria. Journal of Economics and Finance. 1(1), 61- 77.
- Courage, S. O. (2017). 11 Top Crops Farming and production in Nigeria; how to start & make money. Business Classroom for Africans (Since 2007). Retrieved from
https://www.africabusinessclassroom.com/crops-farming-production-nigeria- start-make-money/ on 22 April, 2019
- Degrande, , & Duguma, B., (2000). Adoption Potential of Rotational Hedge Row.
- Intercropping in Humid and Extension Network, 103(1), 1-7
- Diao, X. (2010). Economic Importance of Agriculture for Sustainable Development and Poverty Reduction: Findings from a Case Study of Ghana, Global Forum on Agriculture 29-30 November 2010 Policies for Agricultural Development, Poverty Reduction and Food Security OECD Headquarters, Paris.
- Dim, C. & Ezenekwe, U. (2013). Does Agriculture Matter for Economic Development? Empirical Evidence from Nigeria. Journal of Finance & Economics, 1(1), 61-77.
- Ekine, D. I. & Onu, C. (2018). The impact of agricultural output on economic growth in Nigeria (1981-2015). IOSR Journal of Economics and Finance, 4(1), 10-14
- El-Gizawy, N. (2016). Agronomy III (Crop Production). New York: Mc Craw Hill Companies.
- Enu, P. (2014). Analysis of the agricultural sector of Ghana and its economic impact on economic growth. Academic Research International, 5(4), 267-277
- Ewetan O., Fakile, N., Urhie, E. & Oduntan E. (2017). Agricultural output and economic growth in Nigeria. Journal of African Research in Business & Technology, 3(1), 1-11.
- FAO (2019a). Ghana at a glance. Food and Agriculture Organisation of the United Nations. Retrieved from http://www.fao.org/ghana/fao-in-ghana/ghana-at-a- glance/en/ on September 20, 2018.
- FAO (2019b). Programmes and Projects. Food and Agriculture Organisation of the United Nations. Retrieved from http://www.fao.org/nigeria/programmes-and- projects/programmes/en/on September 20,
- FAOSTAT (2013). A database of the Food and Agriculture Organization of the United Nations (FAO). Retrieved from http://faostat.fao.orgon September 20, 2018.
- Fleming, M. (1958). External economics and the doctrines of balance growth. The Economic Journal,24(1), 1-14.
- Food and Agricultural Organisation-FAO (2018). Countries by commodity, Food and Agricultural Organisation of the United Nations. UK: FAO Departments and Offices.
- Friedman M. (1980). Memorandum: Response to Questionnaire on monetary policy in treasury and civil service committee memoranda on monetary policy. Journal of Development Economics, 8(3), 55-61.
- Garvelink, .J. Wedding, K. & Hanson, S. (2012). Smallholder Agriculture: A Critical Factor in Poverty Reduction and Food Security in Africa. Washington, D.C.: Center for Strategic and International Studies.
- Gerald, G. (2000). Application to economics: Leontief Model. Groningen: University of Groningen Press.
- Greyling, J.C. (2012). The Role of the Agricultural Sector in the South African Economy (A Thesis). Department of Agricultural Economics, University of Stellenbosch.
- Gujarati, D.N & Porter, D.C. (2009). Basic Econometrics (5th). New York: Mc Craw Hill Companies.
- Hanjra, M.A. & Culas, R.J. (2011). The political economy of maize production and poverty reduction in Zambia. Analysis of the last 50 years. Journals of Asian and African Studies, 46(6), 546-566.
Author's Affiliation
Joseph M. IBBIH1, Jorji Akudo NWOGU2
1Department of Economics, Faculty of Social Sciences, Nassarawa State University, Keffi Nigeria
2Department of Economics, Federal College of Education (Technical) Gusau, Zamfara State, Nigeria
Article Details
- Issue: Vol 4 No 5 (2024): Volume 04 Issue 05 May 2024
- Page No.: 348-360
- Published : 10 May 2024
- DOI: https://doi.org/10.55677/ijssers/V04I5Y2024-02
How to Cite :
Impact of Crop Production on Economic Growth in Nigeria, 1981 – 2018. Joseph M. IBBIH, Jorji Akudo NWOGU , 4(5), 348-360. Retrieved from https://ijssers.org/single-view/?id=9598&pid=9590
HTML format
0
View
130
Copyrights & License
This work is licenced under a Creative Commons Attribution 4.0 International License.
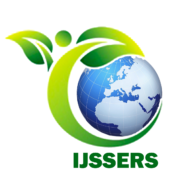
International Journal of Social Science and Education Research Studies
Main Menu
