Estimation of Granger-Causality in the Nigerian Cattle Market
1Y. M. Bulama, 2C. O. Ojo, 3Y. Bila
1,2,3Department of Agricultural Economics, University of Maiduguri, Nigeria.
ABSTRACT *Published Online: 06 January 2022 | |
The research investigated Granger-causality in the Nigerian cattle market. The test was conducted for 45 market pairs. Multi-stage and simple random sampling techniques were used to select two states each from five out of the six geo-political zones in Nigeria, except South-East zone which was not represented due to unavailability of data. A total of ten states were selected. Data were analysed using Granger-Causality test. The Granger causality test result showed less than half of the 45 market pairs studied indicated presence of causality, such that, 16 pairs showed unidirectional price causations and two pairs showed bi-directional price causations, which implies poor price transmission system. Only one market (Zamfara) showed strong links to the other markets. The prices also tended to be supply-driven rather than demand driven, as shown by the prices of Edo, Cross-River and Osun. There is need to improve on the market information system and transportation and infrastructural facilities in order to ensure a good and efficient market and pricing system in the country. |
Keywords: Granger-causality, Nigerian cattle market, Price transmission |
INTRODUCTION
Marketing is an economic activity which stimulates further production and if efficiently done, both the producer and consumer get satisfied in the sense that the former gets a sufficiently remunerative price for the product to continue to produce while the latter gets it at an affordable price that stimulates continued consumption (Mafimisebi et al., 2013).
Livestock markets, in most developing countries like Nigeria, are generally under the control of local authorities. The markets are usually not fenced, they lack essential facilities such as feed and water infrastructure, there are no permanent animal routes, no standard scales (trading is done by ‘eye-ball’ pricing) and remote markets lack price information. Generally, the main actors involved in cattle trading are the local farmers and rural traders, small traders, large traders and butchers. Other actors are the Gainako (herds-boy), Dillalai (Commission agents) and transporters. The local farmers and rural traders transact at farm level with very minimal volume (1-2 animals per transaction). They sell to small specialized traders (who bring cattle from different corners to local/village markets) and to large traders (who transact with butchers on some number of animals mainly for
slaughter at regional/secondary markets.
*Corresponding Author: Y. M. Bulama
*Cite this Article: Y. M. Bulama, C. O. Ojo, Y. Bila (2022). Estimation of Granger-Causality in the Nigerian Cattle Market. International Journal of Social Science and Education Research Studies, 2(1), 15-21
Others are transported to other terminal markets in other parts of the country by the large traders (Bulama, 2004; Addis and Cinda, 2015). Livestock is moved in general purpose vehicles (large trucks) from the north to satisfy the major demand areas of the south in Nigeria (Growth and Employment in States 1, GEMS1, 2012).
Cattle which is important and highly regarded by the people of Nigeria, is largely (about 90%) concentrated in the northern region. This is due to the northern ecological condition characterized by low rainfall, sandy soils and longer dry seasons that provides favourable condition for animal pastoralism. The ecological condition of the north is also unfavourable to the growth of Tsetse fly which is a major disease causing vector of cattle (Lawal-Adebowale, 2012). Therefore, cattle is moved from the north (where it is in surplus) to the south (where it is in shortage) to ensure supply of meat and other cattle products, to consumers in the south. This is done through the process of arbitrage. Since the movement of cattle involves stages, intermediaries and stakeholders, prices of cattle also change at each stage. Due to the considerable spatial separation of production area from consumption area and other factors such as bad roads, there is high handling cost especially in relation to cattle transportation (Mafimisebi et al., 2013). The aim of market integration analysis therefore is to find the possibility of achieving some gains by trading across commodity markets, exploiting price movements in one market (urban) for the prediction of price movements in another market (rural)
The integration of agricultural commodity market is a necessity for the effectiveness of agricultural marketing reform programmes. It ensures the transmission of price signals from food deficit to food surplus areas and help farmers to increase specialization and harness comparative advantages and gains from trade (Okoh and Egbon, 2005). Therefore, there exists a possibility that a price change in one market would result in a series of price responses that spread throughout contiguous market areas (Akintunde et al., 2012). Market integration can be thought of in terms of the long run and the short run. Long-run market integration is where there exists a long-run and stable price relationship between two markets, even if this long-run relationship is disturbed in the short run. Short-run integration is defined as where price changes in a market in one period are passed “immediately” to another market. This pass- through reflects the sensitivity of the price response between markets (Okoh and Egbon, 2005). Two markets are assumed to be integrated if price changes in one market is reflected in the other market. The presence of integration between markets suggests a long-term relationship between the markets. It is assumed that in this long-run relationship there is a direction of price formation in the market system, or the Granger-causality. The Granger-causality is an aspect of market integration studies which determines whether the prices in a market are supply-driven or demand-driven. This is the main focus of this study.
METHODOLOGY
This study made use of secondary data which was obtained from the National Bureau of Statistics (NBS, 2018). Quarterly prices of live cattle collected over a period of 16 years, from first quarter January 2002 to fourth quarter December 2017 (that is, 68 quarters) was used for the analysis.
Nigeria has six-geopolitical zones, with some particular number of states representing each zone, a total of which makes the 36 states in the country. Through a two-stage sampling, the zones were first divided into two: major producing and major consuming zones; the northern states represent the major producing zones while the southern states represent the major consuming zones. Two states were randomly selected from each zone (except for the South East where there was no available data) making a total of 10 states. The states are: Borno and Yobe in the North East: Kano and Zamfara in the North West: Plateau and Nassarawa in the North Central (all producing states): Osun and Oyo in the South West; and Edo and Cross- River in the South South (all-consuming states). The selection according to zones was done to check linkages between cattle markets among zones.
Granger causality was used to test for causality and price transmission between market pairs. The Granger causality technique has improved on the Ravallion model (Ravallion, 1986) and employs a single equation ECM to test for causality between prices. It measures the extent to which current and past price changes in one market explain price changes in another (Hossain and Verbeke, 2010; Rufino, 2011). That is the existence of causality between the two markets in at least one direction (that is, from one market to another), or from the other market to the other, (that is, a two-way causation, feedback) between the markets. If the markets are found to be integrated, then the price in one market will normally be found to Granger-cause the price in the other market (Granger, 1988). The error correction model holds that if the price of a local market and the price of the central market are co-integrated, then the error term from the co-integrating equation should be included, otherwise a first differencing regression between the two prices will be mis-specified and cannot be used to test for market integration (Engle and Granger, 1987)
The implicit assumption of Johansen’s co-integration test is that price adjustments are symmetric, but prices do not stick to one direction in most cases therefore Granger Causality was used to determine if the cattle prices moved in the same direction or otherwise. When multivariate time series are found to be co-integrated, it is important to determine the causal effect of the time series. The co-integration test is incapable of showing the direction of causation between variables (prices), and this necessitates the use of the Granger causality test. Granger (1969) made the first attempt to test for the direction of causality in time series. Granger sought to find whether X causes Y and how much of the current Y can be explained by past values of Y and then see whether adding lagged values of X can give more explanation to the situation. That if X helps in the prediction of Y, then Y is said to be Granger caused by X, and it is a two-way causation, when X Granger-causes Y and Vice Versa.
Hamilton (1994) further explained that if a variable Y fails to Granger cause another variable X, for the set of observations n>0, then the Mean Squared Error (MSE) of a forecast of X 1+n based on lagged values Xt, Xt-1 and Yt, Yt-1 and would be written as:
MSE [Ê (Xt+s| Xt, Xt-1,…,)] = MSE[Ê (Xt,s| Xt, Xt-1,…,Yt, Yt-1)] ———— 1
Where Xt and Yt represent price series of markets X and Y, respectively.
Then X is considered the exogenous variable with respect to Y.
Granger causality was used to test the causality relationship between the cattle market pairs. The model is implemented within a vector auto-correlation regression framework. The test involves estimating the bivariate regressions for all possible pairs of (X, Y) series. The cattle price of one market is regressed on the lagged values of the price in another cattle market. The test is an inference of lead/lag relationship between price of markets and stands to point out whether the lead/lag relationship between two markets is statistically significant or not. The error correction model for estimating Granger Causality is written as:
Pt = tmiPi (t-1) + i=1njPj (t-1) + ɛt ——— 2
Where m and n are numbers of lags (by Akaike information criteria (AIC) for i and j, respectively.
i and j are coefficients to be estimated;
Pt are the prices at time t;
Pi (t-1) and Pj (t-1) are lagged values of prices in markets i and j, respectively.
The null hypothesis is that Pi does not granger cause Pj so that j =0 for j= 1, 2,…, n and i=0, for i= 1, 2,…, n. The null hypothesis is rejected when p value from the chi-statistics (F-test) is greater than 5%. Meaning X cannot influence Y and there is no shot-run causation between X and Y. Rejection of the null hypothesis indicates that market j Granger-Cause price in market i. If price in i also Granger-Cause price in j, the price determination is through a Simultaneous Feedback Mechanism (SFM), that is bi – directional. If Granger-Causality runs one way, it is called unidirectional and the market which leads the other is tagged as the exogenous market. The strength and direction of Granger causality can change over time. And Granger causality means that a lead-lag relationship between variables in a multivariate time series is evident (Granger, 1988).
Four conditions are possible under the Granger-Causality Test:
- Unidirectional causality from X to Y, is indicated if the estimated co-efficient on the lagged X is greater than Zero; that is, i 0 and the estimated coefficients on the lagged Y is equal to Zero, j =0;
- Unidirectional causality from Y to X exist if estimated coefficient on the lagged X is equal to zero, i =0, while estimated coefficient of the lagged Y is not equal to zero, j 0;
- Feedback or bi-directional causality exists, when sets of X and Y coefficients are equal to zero in both regressions.
Independence case is when the sets of X and Y coefficients are not statistically significant, or greater than zero in either of the regressions.
RESULT AND DISCUSSIONS
Causality is the relationship between prices in two markets and shows the direction of price transmission between the two markets. The Granger causality test was applied to the cattle price series and estimated for 45 pairs of cattle markets. The summary of the result of the Granger causality is presented on Table 1.
The result on Table 1 which is inferred from the F-statistics shows there were 16 unidirectional Granger causality transmissions and two bi-directional Granger Causality situations of the 45 pair-wise relationships. Therefore, the null hypothesis for no causality in 25 relationships was accepted, while the alternative was accepted for the pairs that showed the presence of causality. See Appendix for full result.
Table 1: Summary of Granger Causality Test
From Market A | To
Borno |
Market
Cross River |
B
Edo |
Kano | Nassarawa | Osun | Oyo | Plateau | Yobe |
Borno | |||||||||
Cross- River | |||||||||
Edo | → | ||||||||
Kano | ← | ||||||||
Nassarawa | → | ||||||||
Osun | → | ||||||||
Oyo | → | ← | → | → | |||||
Plateau | ← | ←
→ |
|||||||
Yobe | ← | ← | ← | ← | |||||
Zamfara | → | → | →
← |
→ |
Source: Drawn from computed result, 2018 (NBS, 2018)
Note: Markets A= transmitting markets; Markets B= receiving markets
→ = Direction of movement from B to A; = Direction of movement from A to B
= both B to A and A to B
The result on Table 1shows that price changes in Borno are Granger – caused by the price changes in Edo, Nassarawa, Oyo and Zamfara markets. Borno is a surplus region it is
expected that the granger causality goes from Borno to the deficit regions like Edo and Oyo. Edo and Oyo markets are in deficit regions with high demand of cattle which makes them price leaders in this case (due to high demand). This suggests inefficiency in system because prices are formed towards the end of the chain (in the receiving markets) and passed on to the top of the chain (in the supplying markets). Nassarawa and Zamfara are also in surplus regions, therefore the price leadership is expected. This suggests efficiency, since according to theory and practice, goods are transferred from surplus to deficit regions with the supplying region setting prices and taking the power of market leadership.
Price changes in Cross-River Granger – cause price changes in Yobe, while Osun prices Granger – cause Cross-River prices, since Cross-River is in a deficit and demanding region, it may detect prices in Yobe which is a supplying market. Osun is in a region which is more deficit than Cross-River therefore, it may detect prices. This happens when prices are demand –driven.
Edo price changes Granger-cause Kano and Osun price changes. This suggests that Edo leads the price formation process for Borno, Kano and Osun. However, prices changes in Edo are Granger-caused by Zamfara prices. This is possible since Zamfara is in a producing, supplying and surplus region. Price changes in Oyo Granger-cause price changes in Kano in a unidirectional relationship. Oyo is in a deficit or demanding region, and the price formation followed the direction of demand.
Price changes in Kano and Zamfara occurs in a two-way causation, such that prices in Kano Granger – cause prices in Zamfara and vice-versa. This shows a strong relationship between the two markets. Both markets are in supplying (surplus) regions, therefore the bi-directional relationship is expected and also indicates strong price formation and transmission between the two markets.
There are four one way relationships between Nassarawa, Oyo, Osun and Yobe markets. Nassarawa prices Granger – cause price changes in Plateau and Yobe while Osun and Oyo prices Granger – cause price changes in Nassarawa. Nassarawa is in a surplus (and producing) region and produces more than Plateau and Yobe, and becomes the price leader for Plateau and Yobe, in this case. Osun and Oyo are in major deficit regions but have become price leaders in this chain due to high demand. Thus, uni-directional causality implies leader-follower relationship. Plateau and Osun prices Granger–cause each other in a bi-directional relationship. This is the expectance between deficit (Osun) and surplus (Plateau) regions. This indicates good price transmission signals implying efficiency. Zamfara prices Granger–cause price changes in Oyo. This is probably because Zamfara is in a major supplying and surplus region, while Oyo is in a demanding region. Plateau prices Granger-cause price changes Yobe in a one-way causation, though both are in the supplying and producing region, but Plateau prices are more stable.
The result revealed unidirectional causality from Edo, Nassarawa, Oyo and Zamfara to Borno. There is a unidirectional causation from Osun to Cross-River and Cross-River to Yobe. Uni- directional causalities occured from Edo to Kano and Oyo and from Zamfara to Edo, Oyo and Kano. Most of these uni-directional causalities are demand driven rather than supply driven, this is a sign of poor price transmission and inefficiency in the system. Nassarawa is in a major surplus region and has a uni-directional causality towards Borno, Plateau and Yobe markets. Only Oyo prices Granger-caused prices in Nassarawa market.
A bi-directional causality exists between Plateau and Osun, and Zamfara and Kano. Zamfara which is in a surplus and producing region could be termed a price leader for all the other markets since it Granger causes price changes in four markets and only Kano market has any Granger–cause influence on its prices. Yobe could be termed the price taker because it does not Granger–cause price changes in any market while its price changes are Granger–caused by four markets (Plateau, Osun, Nassarawa and Cross-River).
In general, the cattle markets showed weak linkages, since the result showed only 16 uni-directional and two bi-directional relationships out of the 45 market pairs studied. The remaining 25 pairs, which is greater than the total observed causality showed no sign of causality relationship. This weak relationship indicates that there is no dominant market whose price changes influences all other markets suggesting that prices are formed by more than one market. This is probably because of the highly volatile prices that moved together (co-integrated). This weak price transmission which may arise from poor road network and poor price information system existing in most markets, suggests a level of inefficiency in the price system and market as a whole. This also implies lack of coordination and competition in the market system. This is contrary to the findings of Bobola et al., (2015) who discovered 65 bi-directional price relationships of the 91 market pairs studied. The strong link between the market pairs they suggested was due to the relatively stable data and well integrated market prices they studied.
CONCLUSION
The F-statistics for the Granger-Causality test showed there were 16 unidirectional Granger causality transmissions and two bi-directional Granger Causality situations of the 45 pair-wise relationships. Therefore, the null hypothesis for no causality in 25 relationships was accepted. Thus, the study concludes that prices tended to be demand driven rather than supply driven, as shown by the prices of Edo, Cross-River and Osun (major-consuming states). This shows weak price transmission and also indicates that prices are formed and controlled by middlemen leading to lack of competition and poor integration of the markets.
REFERENCES
- Addis, A. B. and Cinda, D. K. (2015). Review of Factors Affecting Livestock Market Price in Lowland Area of Ethiopia. Advances in Life Science and Technology, 37:18-23.
- Akintunde, O.K., Akinremi, T.B., Nwauwa, L.O.E. (2012): Food grain marketing in Osun State, Nigeria: A Study of Long-Run Price Integration. Continental Journal of Agricultural Economics, 6(1):1-9.
- Bobola, O.M., Mafimisebi, T.E., Ikuemonisan, E.S. (2015). Price Fluctuations, Linkages and Causality in the Nigerian Beef Market. Journal of Fisheries and Livestock Production, 3(2): 135-143.
- Bulama, Y. M. (2004). Structure and Performance of Maiduguri Cattle Market, Borno State, Nigeria. Unpublished Master of Science Dissertation Submitted to the School of Post-Graduate Studies University of Maiduguri, Borno State, Nigeria, in Partial fulfillment for the Award of Master of Science Degree in Agricultural Economics.
- Engle, R. E. and Granger, C. W. F. (1987). Co-integration and Error Correction, Representation, Estimation and Testing. Econometrica. 55: 251-276.
- Granger, C. W. (1969). Investigating Causal Relationships by Econometric Models and Cross-Spectral Models. Econometrica, 37 (3): 424-438.
- Granger, C. W. J. (1988). Some Recent Developments in the Concept of Causality. Journal of Econometrics, 30:199-211.
- Growth and Employment in States 1 (GEMS1) (2012). Brief: Transforming the Nigerian Beef Industry. Support to Meat and Leather Industry. World Bank and DFID funded.
- Hamilton, J. D. (1994). Time Series Models of Heteroskedasticity. Time Series Analysis. Princeton University Press, Princeton, New Jersey. pp 657.
- Hossain, M. I. and Verbeke, W. (2010). Evaluation of Rice Markets Integration in Bangladesh. The Lahore Journal of Economics, 15(2): 77-96.
- Lawal-Adebowale, O. A.(2012). Dynamics of Ruminant Livestock Management in the Context of the Nigerian Agricultural System. Chapter 4, INTECH. http://dx.doi.org/10.5772/52923
- Mafimisebi, T. E., Bobola, O. M. and Mafimisebi, O. E. (2013). Fundamentals of Cattle Marketing in Southwest Nigeria: Analyzing Market Intermediaries, Price Formation and Yield Performance. Invited Paper Presented at the Fourth International Conference of the African Association of Agricultural Economists, Hammamet, Tunisia.
- National Bureau of Statistics (2018). Cattle price series: Database.
- Okoh, R.N. and Egbon, P.C. (2005): The integration of Nigeria’s rural and urban foodstuffs markets. African Economic Research Consortium, Nairobi (AERC) Research Paper 151.
- Ravallion, M. (1986). Testing Market Integration. American Journal of Agricultural Economics, 68(1): 45-52.
- Rufino, C.C. (2011). Analyzing the Philippines Inter-Regional Market Integration for Rice. International Research Journal of Finance and Economics, 69: 109-127.
APPENDIX: Pair-wise Granger Causality Test | |||
Date: 02/20/18 Time: 07:20 | |||
Sample: 2002Q1 2017Q4 | |||
Lags: 4 | |||
Null Hypothesis: | Obs | F-Statistic | Prob. |
CROSS_RIVER does not Granger Cause BORNO | 60 | 0.24090 | 0.9138 |
BORNO does not Granger Cause CROSS_RIVER | 0.05558 | 0.9941 | |
EDO does not Granger Cause BORNO | 60 | 2.45670 | 0.0573 |
BORNO does not Granger Cause EDO | 0.04252 | 0.9965 | |
KANO does not Granger Cause BORNO | 60 | 0.49233 | 0.7413 |
BORNO does not Granger Cause KANO | 0.09568 | 0.9834 | |
NASSARAWA does not Granger Cause BORNO | 60 | 2.28414 | 0.0729 |
BORNO does not Granger Cause NASSARAWA | 0.10849 | 0.9790 | |
OSUN does not Granger Cause BORNO | 60 | 0.09317 | 0.9842 |
BORNO does not Granger Cause OSUN | 2.38319 | 0.0635 | |
OYO does not Granger Cause BORNO | 60 | 3.17948 | 0.0208 |
BORNO does not Granger Cause OYO | 0.91435 | 0.4628 | |
PLATEAU does not Granger Cause BORNO | 60 | 0.07166 | 0.9904 |
BORNO does not Granger Cause PLATEAU | 0.16221 | 0.9565 | |
YOBE does not Granger Cause BORNO | 60 | 0.20474 | 0.9346 |
BORNO does not Granger Cause YOBE | 0.03052 | 0.9981 | |
ZAMFARA does not Granger Cause BORNO | 60 | 2.29609 | 0.0717 |
BORNO does not Granger Cause ZAMFARA | 0.04833 | 0.9955 | |
EDO does not Granger Cause CROSS_RIVER | 60 | 0.03229 | 0.9979 |
CROSS_RIVER does not Granger Cause EDO | 0.21949 | 0.9264 | |
KANO does not Granger Cause CROSS_RIVER | 60 | 0.06048 | 0.9930 |
CROSS_RIVERS does not Granger Cause KANO | 0.30068 | 0.8761 | |
NASSARAWA does not Granger Cause CROSS_RIVER | 60 | 0.04302 | 0.9964 |
CROSS_RIVER does not Granger Cause NASSARAWA | 0.26787 | 0.8973 | |
OSUN does not Granger Cause CROSS_RIVER | 60 | 26.9900 | 5.E-12 |
CROSS_RIVER does not Granger Cause OSUN | 0.40303 | 0.8056 | |
OYO does not Granger Cause CROSS_RIVER | 60 | 0.74757 | 0.5642 |
CROSS_RIVER does not Granger Cause OYO | 0.18814 | 0.9435 | |
PLATEAU does not Granger Cause CROSS_RIVER | 60 | 0.26513 | 0.8990 |
CROSS_RIVER does not Granger Cause PLATEAU | 0.24648 | 0.9105 | |
YOBE does not Granger Cause CROSS_RIVER | 60 | 0.33410 | 0.8537 |
CROSS_RIVER does not Granger Cause YOBE | 114.596 | 8.E-25 | |
ZAMFARA does not Granger Cause CROSS_RIVER | 60 | 0.04055 | 0.9968 |
CROSS_RIVER does not Granger Cause ZAMFARA | 0.21744 | 0.9275 | |
KANO does not Granger Cause EDO | 60 | 0.06991 | 0.9908 |
EDO does not Granger Cause KANO | 10.5020 | 3.E-06 | |
NASSARAWA does not Granger Cause EDO | 60 | 0.02171 | 0.9991 |
EDO does not Granger Cause NASSARAWA | 0.01860 | 0.9993 | |
OSUN does not Granger Cause EDO | 60 | 0.08404 | 0.9870 |
EDO does not Granger Cause OSUN | 0.02048 | 0.9992 | |
OYO does not Granger Cause EDO | 60 | 0.06678 | 0.9916 |
EDO does not Granger Cause OYO | 5.87823 | 0.0006 | |
PLATEAU does not Granger Cause EDO | 60 | 0.02964 | 0.9982 |
EDO does not Granger Cause PLATEAU | 0.02942 | 0.9983 | |
YOBE does not Granger Cause EDO | 60 | 0.19542 | 0.9397 |
EDO does not Granger Cause YOBE | 0.01750 | 0.9994 | |
ZAMFARA does not Granger Cause EDO | 60 | 3.06905 | 0.0243 |
EDO does not Granger Cause ZAMFARA | 0.02335 | 0.9989 | |
NASSARAWA does not Granger Cause KANO | 60 | 0.02974 | 0.9982 |
KANO does not Granger Cause NASSARAWA | 0.03323 | 0.9978 | |
OSUN does not Granger Cause KANO | 60 | 0.12753 | 0.9718 |
KANO does not Granger Cause OSUN | 0.03873 | 0.9970 | |
OYO does not Granger Cause KANO | 60 | 18.7896 | 2.E-09 |
KANO does not Granger Cause OYO | 0.11778 | 0.9756 | |
PLATEAU does not Granger Cause KANO | 60 | 0.04213 | 0.9965 |
KANO does not Granger Cause PLATEAU | 0.05409 | 0.9944 | |
YOBE does not Granger Cause KANO | 60 | 0.20089 | 0.9367 |
KANO does not Granger Cause YOBE | 0.03070 | 0.9981 | |
ZAMFARA does not Granger Cause KANO | 60 | 10.4907 | 3.E-06 |
KANO does not Granger Cause ZAMFARA | 3.38638 | 0.0156 | |
OSUN does not Granger Cause NASSARAWA | 60 | 4.80740 | 0.0023 |
NASSARAWA does not Granger Cause OSUN | 0.20557 | 0.9342 | |
OYO does not Granger Cause NASSARAWA | 60 | 2.51054 | 0.0531 |
NASSARAWA does not Granger Cause OYO | 0.07887 | 0.9884 | |
PLATEAU does not Granger Cause NASSARAWA | 60 | 0.04586 | 0.9959 |
NASSARAWA does not Granger Cause PLATEAU | 8.85600 | 2.E-05 | |
YOBE does not Granger Cause NASSARAWA | 60 | 0.23545 | 0.9171 |
NASSARAWA does not Granger Cause YOBE | 3.89339 | 0.0078 | |
ZAMFARA does not Granger Cause NASSARAWA | 60 | 0.02349 | 0.9989 |
NASSARAWA does not Granger Cause ZAMFARA | 0.02757 | 0.9985 | |
OYO does not Granger Cause OSUN | 60 | 0.02634 | 0.9986 |
OSUN does not Granger Cause OYO | 0.97624 | 0.4288 | |
PLATEAU does not Granger Cause OSUN | 60 | 10.8341 | 2.E-06 |
OSUN does not Granger Cause PLATEAU | 4.99743 | 0.0018 | |
YOBE does not Granger Cause OSUN | 60 | 0.23025 | 0.9201 |
OSUN does not Granger Cause YOBE | 28.8988 | 1.E-12 | |
ZAMFARA does not Granger Cause OSUN | 60 | 0.02555 | 0.9987 |
OSUN does not Granger Cause ZAMFARA | 0.08709 | 0.9861 | |
PLATEAU does not Granger Cause OYO | 60 | 0.04818 | 0.9955 |
OYO does not Granger Cause PLATEAU | 0.37663 | 0.8243 | |
YOBE does not Granger Cause OYO | 60 | 0.16026 | 0.9574 |
OYO does not Granger Cause YOBE | 0.77546 | 0.5463 | |
ZAMFARA does not Granger Cause OYO | 60 | 7.16269 | 0.0001 |
OYO does not Granger Cause ZAMFARA | 1.89385 | 0.1258 | |
YOBE does not Granger Cause PLATEAU | 60 | 0.25622 | 0.9045 |
PLATEAU does not Granger Cause YOBE | 3.82786 | 0.0085 | |
ZAMFARA does not Granger Cause PLATEAU | 60 | 0.03818 | 0.9971 |
PLATEAU does not Granger Cause ZAMFARA | 0.03845 | 0.9971 | |
ZAMFARA does not Granger Cause YOBE | 60 | 0.02228 | 0.9990 |
YOBE does not Granger Cause ZAMFARA | 0.19278 | 0.9411 | |
Estimation of Granger-Causality in the Nigerian Cattle Market
1Y. M. Bulama, 2C. O. Ojo, 3Y. Bila
1,2,3Department of Agricultural Economics, University of Maiduguri, Nigeria.
Vol 02 No 01 (2020): Volume 02 Issue 01 January 2022
Article Date Published : 6 January 2022 | Page No.: 15-21
Abstract :
The research investigated Granger-causality in the Nigerian cattle market. The test was conducted for 45 market pairs. Multi-stage and simple random sampling techniques were used to select two states each from five out of the six geo-political zones in Nigeria, except South-East zone which was not represented due to unavailability of data. A total of ten states were selected. Data were analysed using Granger-Causality test. The Granger causality test result showed less than half of the 45 market pairs studied indicated presence of causality, such that, 16 pairs showed unidirectional price causations and two pairs showed bi-directional price causations, which implies poor price transmission system. Only one market (Zamfara) showed strong links to the other markets. The prices also tended to be supply-driven rather than demand driven, as shown by the prices of Edo, Cross-River and Osun. There is need to improve on the market information system and transportation and infrastructural facilities in order to ensure a good and efficient market and pricing system in the country.
Keywords :
Granger-causality, Nigerian cattle market, Price transmissionReferences :
- Addis, A. B. and Cinda, D. K. (2015). Review of Factors Affecting Livestock Market Price in Lowland Area of Ethiopia. Advances in Life Science and Technology, 37:18-23.
- Akintunde, O.K., Akinremi, T.B., Nwauwa, L.O.E. (2012): Food grain marketing in Osun State, Nigeria: A Study of Long-Run Price Integration. Continental Journal of Agricultural Economics, 6(1):1-9.
- Bobola, O.M., Mafimisebi, T.E., Ikuemonisan, E.S. (2015). Price Fluctuations, Linkages and Causality in the Nigerian Beef Market. Journal of Fisheries and Livestock Production, 3(2): 135-143.
- Bulama, Y. M. (2004). Structure and Performance of Maiduguri Cattle Market, Borno State, Nigeria. Unpublished Master of Science Dissertation Submitted to the School of Post-Graduate Studies University of Maiduguri, Borno State, Nigeria, in Partial fulfillment for the Award of Master of Science Degree in Agricultural Economics.
- Engle, R. E. and Granger, C. W. F. (1987). Co-integration and Error Correction, Representation, Estimation and Testing. Econometrica. 55: 251-276.
- Granger, C. W. (1969). Investigating Causal Relationships by Econometric Models and Cross-Spectral Models. Econometrica, 37 (3): 424-438.
- Granger, C. W. J. (1988). Some Recent Developments in the Concept of Causality. Journal of Econometrics, 30:199-211.
- Growth and Employment in States 1 (GEMS1) (2012). Brief: Transforming the Nigerian Beef Industry. Support to Meat and Leather Industry. World Bank and DFID funded.
- Hamilton, J. D. (1994). Time Series Models of Heteroskedasticity. Time Series Analysis. Princeton University Press, Princeton, New Jersey. pp 657.
- Hossain, M. I. and Verbeke, W. (2010). Evaluation of Rice Markets Integration in Bangladesh. The Lahore Journal of Economics, 15(2): 77-96.
- Lawal-Adebowale, O. A.(2012). Dynamics of Ruminant Livestock Management in the Context of the Nigerian Agricultural System. Chapter 4, INTECH. http://dx.doi.org/10.5772/52923
- Mafimisebi, T. E., Bobola, O. M. and Mafimisebi, O. E. (2013). Fundamentals of Cattle Marketing in Southwest Nigeria: Analyzing Market Intermediaries, Price Formation and Yield Performance. Invited Paper Presented at the Fourth International Conference of the African Association of Agricultural Economists, Hammamet, Tunisia.
- National Bureau of Statistics (2018). Cattle price series: Database.
- Okoh, R.N. and Egbon, P.C. (2005): The integration of Nigeria’s rural and urban foodstuffs markets. African Economic Research Consortium, Nairobi (AERC) Research Paper 151.
- Ravallion, M. (1986). Testing Market Integration. American Journal of Agricultural Economics, 68(1): 45-52.
- Rufino, C.C. (2011). Analyzing the Philippines Inter-Regional Market Integration for Rice. International Research Journal of Finance and Economics, 69: 109-127.
Author's Affiliation
1Y. M. Bulama, 2C. O. Ojo, 3Y. Bila
1,2,3Department of Agricultural Economics, University of Maiduguri, Nigeria.
Article Details
- Issue: Vol 02 No 01 (2020): Volume 02 Issue 01 January 2022
- Page No.: 15-21
- Published : 6 January 2022
- DOI:
How to Cite :
Estimation of Granger-Causality in the Nigerian Cattle Market. 1Y. M. Bulama, 2C. O. Ojo, 3Y. Bila, 02(01), 15-21. Retrieved from https://ijssers.org/single-view/?id=6699&pid=6687
HTML format
0
View
142
Copyrights & License
This work is licenced under a Creative Commons Attribution 4.0 International License.
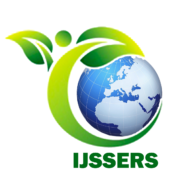
International Journal of Social Science and Education Research Studies
Main Menu
