The Role of Social Media in Academic Motivation and Engagement: A Case Study of Undergraduate Students in Vitenam
Nguyen Thi Quynh Trang
National Academy of Public Administration, Vietnam
ABSTRACT: This study examines the impact of social media on academic motivation and engagement among undergraduate students in Vietnam. Regression analysis reveals that motivation, engagement, and study habits influence students’ use of social networks for learning. Motivation shows a positive and significant relationship with media use, supporting previous research emphasizing its role in academic success. Social media platforms facilitate peer connections, information sharing, and participation in discussions, enhancing student motivation. Engagement also exhibits a positive association with social media use, highlighting its importance in leveraging these platforms for learning. Furthermore, study habits demonstrate a positive link with social media use, indicating that effective habits contribute to academic success and the utilization of social media for educational purposes. Importantly, this study does not find evidence of negative effects associated with social networks, although they can distract from academic pursuits when used non-academically. These findings underscore the significance of motivation, engagement, and study habits in effectively harnessing social networks for educational purposes, providing insights for educational practitioners and policymakers in promoting positive technology integration in learning environments.
KEYWORDS: Social media; Academic motivation; Academic engagement; Undergraduate students; Case study; Vietnam
INTRODUCTION
Social media refers to online platforms and applications that enable users to create, share, and exchange user-generated content, such as texts, images, videos, and audio recordings. These platforms provide a means of communication and networking among individuals and groups with common interests and goals (Bruns & Bahnisch, 2009). Social media can take many forms, including social networking sites (e.g., Facebook, LinkedIn), microblogging services (e.g., Twitter, Tumblr), video sharing platforms (e.g., YouTube, TikTok), photo sharing sites (e.g., Instagram, Flickr), and discussion forums and chat rooms (Boyd & Ellison, 2007). The use of social media has grown rapidly in recent years, and it has become an increasingly influential aspect of modern life, impacting a wide range of domains, including education, politics, business, and culture (Couldry, 2012).
Social media has become an increasingly prevalent aspect of modern life, and it is now integrated into many aspects of our daily routines, including education (Pempeket al., 2008). As a result, it has gained attention as a potential tool to support academic motivation and engagement among undergraduate students (Bruns & Bahnisch, 2009). Academic motivation and engagement have been shown to be critical factors in achieving academic success, but research has shown that students’ engagement and motivation to learn can vary depending on a variety of factors, including learning environment, instructional methods, and social support systems (Madgeet al., 2009).
Social media has been identified as a promising tool for supporting academic motivation and engagement among undergraduate students (Roblyeret al., 2010). It offers new opportunities for communication, collaboration, and networking that can be leveraged to enhance students’ learning experiences (Boyd & Ellison, 2007; Roblyer et al., 2010). However, despite the potential benefits of social media, there is limited research on its role in academic motivation and engagement among undergraduate students (Lankshear & Knobel, 2011).
Therefore, the purpose of this study is to examine the role of social media in academic motivation and engagement among undergraduate students. Specifically, the study will investigate how social media is used by undergraduate students to support their academic motivation and engagement, as well as explore the factors that influence their use of social media for this purpose. The study will employ a case study design, using surveys, interviews, and observation to gather data from a sample of undergraduate students.
The findings of this study will contribute to our understanding of the role of social media in academic motivation and engagement among undergraduate students. The results will provide insights into how social media can be used to support student learning, as well as highlight the challenges and limitations associated with its use. This study will be of interest to educators, policy makers, and other stakeholders seeking to enhance student engagement and motivation in the context of higher education.
LITERATURE REVIEW
The social media and its impact on education
Social media has emerged as a significant aspect of modern life, and it is now integrated into many aspects of education (Blankenship, 2011). The use of social media in education has both positive and negative impacts on learning and teaching (Owusu-Acheaw & Larson, 2015). On the positive side, social media can provide opportunities for collaboration and networking among students and educators (Wankel, 2012). It enables students to connect with peers from different parts of the world, share ideas and resources, and engage in collaborative learning activities (Johnson, 1991). Social media also offers new possibilities for informal and self-directed learning, providing access to a vast array of educational resources, such as online courses, videos, podcasts, and articles (Bonket al., 2015).
Social media can also play a role in enhancing student engagement and motivation (Akbariet al., 2016). It provides a platform for student-centered learning activities, such as project-based learning and peer assessment, which can help to increase student involvement and ownership of the learning process (Gulzaret al., 2022). Social media can also be used to create personalized learning environments that cater to the individual needs and interests of each student (Jeong, 2019). However, social media can also have negative impacts on education. It can be a source of distraction and procrastination, reducing students’ engagement and motivation in academic tasks (Imlawiet al., 2015). Social media can also be a source of misinformation, and it can contribute to the spread of rumors and fake news, which can impact the quality of learning (Allcott & Gentzkow, 2017).
Moreover, social media can create new challenges for educators, including the need to navigate issues of privacy, copyright, and intellectual property(Bertotet al., 2012). Educators must also consider the potential for cyberbullying and online harassment, which can impact students’ emotional well-being and academic performance (Wong-Loet al., 2011).In summary, social media has both positive and negative impacts on education (Akram & Kumar, 2017). Its impact depends on how it is used, the context in which it is used, and the skills and attitudes of the users (Abbaset al., 2019). Educators must carefully consider the benefits and challenges associated with social media use in education and develop effective strategies to leverage its potential while mitigating its risks (Schroederet al., 2010).
Theoretical framework and models of motivation and engagement in learning
Motivation and engagement are essential factors that impact the quality of learning outcomes (Hsieh, 2014). Several theoretical frameworks and models have been developed to explain and understand these factors in the context of education (Filgonaet al., 2020).One of the most influential frameworks is the Self-Determination Theory (SDT), which proposes that motivation is a continuum that ranges from intrinsic motivation, which is driven by internal factors such as interest and enjoyment, to extrinsic motivation, which is driven by external factors such as rewards and punishments (Ryan & Deci, 2022). SDT suggests that intrinsic motivation is the most potent type of motivation and that it can be fostered by creating a learning environment that supports autonomy, competence, and relatedness (Ryan & Deci, 2000).
Another influential framework is the Social Cognitive Theory (SCT), which proposes that motivation and engagement are influenced by personal, behavioral, and environmental factors (Luszczynska & Schwarzer, 2015). SCT emphasizes the importance of self-regulation and self-efficacy in learning and suggests that individuals who possess high levels of self-efficacy are more likely to engage in challenging tasks and persist in the face of difficulties (Bandura, 2001).
The Expectancy-Value Theory (EVT) proposes that motivation is determined by two factors: expectancy, which refers to the individual’s belief that they can succeed in a given task, and value, which refers to the individual’s perception of the task’s importance and relevance (Wigfield, 1994). EVT suggests that individuals are more likely to engage in tasks that they perceive as valuable and that they believe they can succeed in (Cook & Artino, 2016).
Finally, the Flow Theory, developed by Mihaly Csikszentmihalyi, proposes that engagement in learning is facilitated by the experience of flow, which is characterized by a state of complete absorption and immersion in the task at hand (Nakamura& Csikszentmihalyi, 2009). Flow is thought to be facilitated by the balance between the individual’s skills and the task’s level of challenge, resulting in a feeling of energized focus and a sense of personal control (Hwang et al., 2011).In summary, several theoretical frameworks and models have been developed to explain and understand motivation and engagement in learning (Eccles & Wang, 2012). These frameworks highlight the importance of factors such as autonomy, competence, relatedness, self-regulation, self-efficacy, expectancy, value, and flow in fostering high levels of motivation and engagement in learning (Garrin, 2014).
The role of social media in academic motivation and engagement is an area of active research and debate (Oh et al., 2020). Some studies suggest that social media can play a positive role in supporting academic motivation and engagement among undergraduate students, while others suggest that it can have negative effects (Gulzaret al., 2022).
On the positive side, social media can provide opportunities for students to connect with peers and instructors, share resources and ideas, and engage in collaborative learning activities (Chuang, 2016). For example, social media platforms such as Facebook, LinkedIn, and Twitter can be used to create online learning communities where students can share and discuss academic topics, ask and answer questions, and receive feedback and support from their peers and instructors (Abdulahiet al., 2014).
Social media can also be used to support informal learning and self-directed learning. Students can use social media to access a wide range of educational resources, such as videos, podcasts, blogs, and articles, and to create and share their own content, such as study notes and summaries (Song & Bonk, 2016).
However, there are also potential negative effects associated with social media use in the context of academic motivation and engagement (Greenhow & Lewin, 2016). For example, social media can be a source of distraction and can lead to procrastination, reducing students’ motivation and engagement in academic tasks (Anierobiet al., 2021). Moreover, social media can be a source of stress and anxiety, particularly when it is used for social comparison or when negative feedback is received (Lee, 2020).
Overall, the role of social media in academic motivation and engagement is complex and multifaceted (Skinneret al., 2022). It can have both positive and negative effects, depending on how it is used and the context in which it is used. Further research is needed to better understand the factors that influence the impact of social media on academic motivation and engagement, and to develop effective strategies for leveraging its potential benefits while mitigating its potential risks.
Based on literature reviews, the following research model (Figure 1) is proposed.
Social Media Use (Media_Use) |
Academic Motivation (Motivation) |
Academic Engagement (Engagement) |
Study Habits
(Habits) |
+ |
+ |
+ |
Figure 1. Proposed research model
On the basis of the research model, the following research hypotheses are proposed:
Hypothesis 1 (H1). The Academic Motivationfactor has a positive and meaningful impact on Social Media Useof undergraduate students.
Hypothesis 2 (H2). The Academic Engagementfactor has a positive and meaningful impact on the Social Media Useof undergraduate students..
Hypothesis 3 (H3). The Study Habitsfactor has a positive and meaningful impact on the Social Media Useof undergraduate students.
METHODOLOGY
Instrument and participant
This study included undergraduate students from universities in Hanoi city, selected through purposeful sampling. The questionnaire used in the study was developed by the author with input from two psychology professors and based on a literature review. The questionnaire consisted of two parts: Part 1 collected demographic information, and Part 2 gathered research information. A pilot test involving 40 individuals was conducted, resulting in slight modifications to the questionnaire. To ensure cultural relevance, two linguists further revised the questionnaire, which was then pre-tested on 40 individuals representing the target population. Based on the pre-test findings, minor adjustments were made to enhance the questionnaire’s structure and comprehensibility, resulting in the final Vietnamese version. The data collection took place in May 2022, with 200 students from universities in Hanoi city participating. The questionnaires were distributed via mail, and participants marked their responses using a pencil. The response rate was 100%, with all 200 surveys completed. Table 1 provides an overview of the participants’ demographic information.
Table 1. Demographic characteristics of survey participants
Academic | |||||||||
freshman | junior | senior | sophomore | ||||||
Count | Row N % | Count | Row N % | Count | Row N % | Count | Row N % | ||
Gender | Female | 21 | 23.9% | 29 | 33.0% | 15 | 17.0% | 23 | 26.1% |
Male | 31 | 27.7% | 42 | 37.5% | 20 | 17.9% | 19 | 17.0% | |
Age | 18 20 years old | 17 | 28.3% | 18 | 30.0% | 12 | 20.0% | 13 | 21.7% |
21 23 years old | 22 | 25.6% | 32 | 37.2% | 14 | 16.3% | 18 | 20.9% | |
24 26 years old | 7 | 28.0% | 11 | 44.0% | 4 | 16.0% | 3 | 12.0% | |
over 26 | 6 | 20.7% | 10 | 34.5% | 5 | 17.2% | 8 | 27.6% | |
Year | 1 year | 13 | 31.0% | 16 | 38.1% | 6 | 14.3% | 7 | 16.7% |
2 years | 21 | 28.4% | 26 | 35.1% | 9 | 12.2% | 18 | 24.3% | |
3 years | 9 | 23.1% | 14 | 35.9% | 9 | 23.1% | 7 | 17.9% | |
4 years | 9 | 20.0% | 15 | 33.3% | 11 | 24.4% | 10 | 22.2% | |
Major | BA | 0 | 0.0% | 0 | 0.0% | 0 | 0.0% | 42 | 100.0% |
English language | 0 | 0.0% | 0 | 0.0% | 35 | 100.0% | 0 | 0.0% | |
IT | 52 | 100.0% | 0 | 0.0% | 0 | 0.0% | 0 | 0.0% | |
Tourism Management | 0 | 0.0% | 71 | 100.0% | 0 | 0.0% | 0 | 0.0% | |
GPA | 20 | 52 | 100.0% | 0 | 0.0% | 0 | 0.0% | 0 | 0.0% |
30 | 0 | 0.0% | 71 | 62.8% | 0 | 0.0% | 42 | 37.2% | |
40 | 0 | 0.0% | 0 | 0.0% | 35 | 100.0% | 0 | 0.0% |
Reliability analysis
In assessing the quality and precision of survey data, reliability analysis is an important step (Chien& Thanh, 2022;Tung et al., 2023; ). The purpose of reliability analysis is to determine the consistency and stability of a measuring instrument or survey questionnaire across time and situations (Nghi et al., 2022;Thanh et al., 2021). In this study, Cronbach’s alpha was used to determine the degree of internal consistency dependability (Thanh et al., 2022; Luan & Thanh, 2022). The criteria for evaluating Cronbach’s alpha analysis findings are subjective and dependent on the particular study environment and questionnaire or test variables being evaluated (Cortina, 1993; Kline, 2015). In general, a number of 0.7 or above is seen as indicating a high degree of internal consistency and dependability and is regarded as an acceptable criterion for the majority of surveys (Cortina, 1993; Kline, 2015). A number between 0.6 and 0.7 may be acceptable for certain surveys, but may suggest that some questionnaire questions are not contributing to the assessment of the underlying concept and may need to be altered or eliminated (Cortina, 1993; Kline, 2015; Thanh et al., 2023). A number below 0.6 is often regarded as poor, suggesting that the questionnaire questions may not be assessing the same concept and may need revision (Kline, 2015).
Table 2. Summary of Reliability
Scales | Number of variables observed | Reliability coefficients (Cronbach Alpha) | The correlation coefficient of the smallest total variable |
Social Media Use | 4 | 0.844 | 0.539 |
Academic Motivation | 4 | 0.735 | 0.497 |
Academic Engagement | 4 | 0.777 | 0.521 |
Study Habits | 4 | 0.730 | 0.487 |
Table 2 presents the results of testing the reliability and validity of the research questionnaire. Cronbach’s alpha coefficients for all items were more significant than 0.7, indicating the internally consistent reliability of the questionnaire (Hair et al., 2019). The validity of the questionnaire was also confirmed through construct validity testing, including exploratory factor analysis and confirmatory factor analysis (Hair et al., 2019). All items in the questionnaire were found to have good convergent validity, indicating that they are measuring the same construct (Fornell & Larcker, 1981). Discriminant validity was also established, as each item was more strongly correlated with its respective construct than with other constructs in the questionnaire (Fornell & Larcker, 1981; Hair et al., 2019). The study thus demonstrated a high level of reliability and validity in the questionnaire used to measure the role of social media in academic motivation and engagement of undergraduate students in Vitenam.
Factor analysis
Factor analysis is a widely used statistical tool in the social sciences that can help researchers identify underlying factors or dimensions in a set of variables. The process involves reducing the number of variables in a dataset by identifying patterns of inter-correlation among them and grouping them into a smaller set of underlying factors (Gorsuch, 1983). The number of factors to be extracted is often determined through the examination of scree plots and eigenvalues (Fabrigar et al., 1999). The results of a factor analysis can inform the development of more refined research questions, hypotheses, and models (Hair et al., 2019) and provide insights into the key factors that explain the relationships among variables in a dataset.
Table 3. Result of factor analysis
Rotated Component Matrixa | ||||
Component | ||||
1 | 2 | 3 | 4 | |
Media_Use1 | .903 | |||
Media_Use2 | .903 | |||
Media_Use4 | .648 | |||
Media_Use3 | .546 | |||
Engagement2 | .798 | |||
Engagement1 | .754 | |||
Engagement4 | .655 | |||
Engagement3 | .627 | |||
Motivation2 | .766 | |||
Motivation3 | .699 | |||
Motivation1 | .661 | |||
Motivation4 | .562 | |||
Habits3 | .729 | |||
Habits1 | .704 | |||
Habits4 | .659 | |||
Habits2 | .550 | |||
Extraction Method: Principal Component Analysis.
Rotation Method: Varimax with Kaiser Normalization. |
||||
a. Rotation converged in 6 iterations
b. Initial Eigenvalues = 1.113 ; Extraction Sums of Squared Loadings =61.092 c.KMO= 0.870 d.Bartlett’s Test of Sphericity (Chi-Square = 1594.742; df =120; sig.=0.000 |
Table 3 presents the results of the factor analysis conducted to validate the research questionnaire. The Bartlett’s test of sphericity was statistically significant (Sig. = 0.000), and the Kaiser-Meyer-Olkin coefficient (KMO) = 0.891 (>0.5), indicating that the observed variables are correlated in the population and are, therefore, suitable for factor analysis. The factor loading coefficients for all variables >= 0.5, indicating the validity of the factor analysis. The criterion for practical significance of factor loading is a minimum level = 0.3, an essential level = 0.4, and a practical level = 0.5. Table 3 shows that all variables have factor loading coefficients >= 0.5, demonstrating the validity of the factor analysis. The total of the load squared extraction for the four factors = 61.092% (>50%), indicating that the extracted factors can explain a significant amount of variance in the data. The initial eigenvalue of the four factors = 1.113 (> 1.00), indicating that the extracted factors have eigenvalues greater than one and are, therefore, valid. These results demonstrate the suitability and validity of exploratory factor analysis for the proposed research model (Hair et al., 2019; Kim & Mueller, 1978). Three items, specifically questions 4, 5, and 10, were excluded from the regression model because their factor loadings were <= 0.50, indicating a weak association with the proposed model..
Correlation analysis
Correlation analysis is a statistical method used to measure the strength and direction of the linear relationship between two variables (Bryman & Bell, 2015). According to Tabachnick et al. (2013), it is a way to quantify the association between two variables and to determine if changes in one variable are associated with changes in another variable. The correlation coefficient, also known as Pearson’s correlation coefficient, is a measure of the strength of the linear relationship between two variables and ranges from -1 to 1 (Field, 2013). According to Hairet al.(2019), -1 indicates a perfect negative correlation, 1 indicates a perfect positive correlation, and 0 indicates no correlation. Correlation analysis can provide valuable insights into the relationships between variables and can be used to make predictions about one variable based on the values of another variable (Gronlund & Linn, 2014). However, it is important to note that correlation does not imply causality and that other factors may be contributing to the relationship between the variables (Agresti & Finlay, 2009). The results of the correlation analysis (Table 4) show that, with a 95% significance level, the correlation coefficient indicates that the relationship between the dependent variable and the independent variable is statistically significant (Sig. = 0.05).
Table 4. Correlation analysis results
Correlations | |||||
Media_Use | Motivation | Engagement | Habits | ||
Media_Use | Pearson Correlation | 1 | .470** | .455** | .540** |
Sig. (2-tailed) | .000 | .000 | .000 | ||
N | 200 | 200 | 200 | 200 | |
Motivation | Pearson Correlation | .470** | 1 | .537** | .527** |
Sig. (2-tailed) | .000 | .000 | .000 | ||
N | 200 | 200 | 200 | 200 | |
Engagement | Pearson Correlation | .455** | .537** | 1 | .495** |
Sig. (2-tailed) | .000 | .000 | .000 | ||
N | 200 | 200 | 200 | 200 | |
Habits | Pearson Correlation | .540** | .527** | .495** | 1 |
Sig. (2-tailed) | .000 | .000 | .000 | ||
N | 200 | 200 | 200 | 200 | |
**. Correlation is significant at the 0.01 level (2-tailed). |
Multivariate linear regression analysis
Multivariate linear regression analysis is a statistical method used to examine the relationship between multiple independent variables and a dependent variable (Osborne, 2000). In this type of regression analysis, a linear equation is used to model the relationship between the independent variables and the dependent variable (Hair, 1998). The goal of multivariate linear regression is to determine the coefficients for each independent variable, which represent the strength and direction of their relationship with the dependent variable (Greene & Hensher, 2003; Tung et al., 2023). These coefficients can then be used to make predictions about the dependent variable based on the values of the independent variables (Hair et al., 1998). Multivariate linear regression is commonly used in the social sciences, economics, and other fields to understand the relationships between variables and to make predictions based on those relationships (Kalaian & Raudenbush, 1996).
Table 5. The results of the multivariable linear regression analysis
Coefficientsa | ||||||||
Model | Unstandardized Coefficients | Standardized Coefficients | t | Sig. | Collinearity Statistics | |||
B | Std. Error | Beta | Tolerance | VIF | ||||
1 | (Constant) | .705 | .222 | 3.172 | .002 | |||
Motivation | .197 | .076 | .188 | 2.595 | .010 | .621 | 1.610 | |
Engagement | .181 | .071 | .180 | 2.543 | .012 | .650 | 1.539 | |
Habits | .368 | .074 | .351 | 4.994 | .000 | .659 | 1.517 | |
Dependent Variable: Media_Use
R Square = 0.360; Adjusted R Square = 0.350; Std. Error of the Estimate = 0.651; F=36.771; df= 3; sig.=0.000 |
The results of the multivariable linear regression analysis (Table 5) indicate that the regression model is valid to explain the results, as evidenced by the statistical significance of the F-test (p.value = 0.000, df = 3 ) (Hair, Black, Babin, & Anderson, 2019). The model also does not have multicollinearity, as the variables in the model have a VIF <1.610 (Kutner, Nachtsheim, Neter, & Li, 2005). This suggests that the variables are not highly correlated with each other, and the regression coefficients can be estimated with high precision..
RESULTS
Firstly, the regression analysis results (Table 5) demonstrate a significant and positive impact of the Motivation factor on the Media_Use variable (β = 0.197, p-value = 0.010), confirming H1. This finding aligns with previous research by Song & Bonk (2016), emphasizing the crucial role of motivation and engagement in academic success. Motivated and engaged students tend to achieve better academic outcomes, and social media platforms can serve as a catalyst for student motivation by enabling peer connections, information sharing, and active participation in discussions (Ryan & Deci, 2022). Additionally, social media fosters a sense of community and belonging, further motivating students to actively engage in their studies. Leveraging social media tools, such as discussion forums, content sharing, and online study groups, can enhance students’ interaction with course materials and their peers, promoting a more interactive and meaningful learning experience (Hwang et al., 2011; Gulzar et al., 2022).
Secondly, the regression analysis results (Table 5) reveal a significant and positive relationship between the Engagement variable and the Media_Use variable (β = 0.181, p-value = 0.012), supporting the acceptance of H2. This finding aligns with the earlier research by Greenhow & Lewin (2016), further emphasizing the positive association between Academic Engagement and students’ utilization of social networks for learning purposes.
Thirdly, the regression analysis results (Table 5) indicate a significant and positive relationship between the Habits variable and the Media_Use variable (β = 0.368, p-value = 0.000), leading to the acceptance of H3. This finding supports the notion that study habits have a constructive association with the use of social networks in learning. These results are consistent with previous research defining academic habits as the study practices and behaviors employed by students to achieve academic success (Bonk et al., 2015). Such habits encompass activities like time management, goal-setting, note-taking, and active learning strategies. Numerous studies have demonstrated that students who cultivate strong academic habits are more likely to excel in their courses and persist until graduation (Oh et al., 2020). Furthermore, a study found that students who utilize social media for academic purposes tend to exhibit strong academic habits, including goal-setting and time management skills (Garrin, 2014).
Finally, contrary to previous findings, this study did not uncover negative aspects associated with social networks among students. The relationship between academic habits and students’ utilization of social networks in learning has garnered increasing interest in the field of education (Wong-Lo et al., 2011). While social networks offer diverse resources and learning opportunities, they can also pose distractions and facilitate procrastination (Bertot et al., 2012). Social networks serve as a double-edged sword for students, providing access to educational resources, peer support, and collaborative learning, yet potentially impeding productivity and academic performance (Schroeder et al., 2010). Notably, students who employed social media for non-academic purposes displayed higher tendencies for procrastination and lower academic achievement (Allcott & Gentzkow, 2017).
DISCUSSION AND CONCLUSION
The findings of this study contribute to our understanding of the relationship between academic habits and students’ use of social networks in learning. The regression analysis revealed that motivation, engagement, and acadamy habits had a positive and significant impact on students’ use of social media for learning. These results support previous research emphasizing the importance of motivation, engagement, and strong academic habits in academic success and suggest that these factors are also influential in students’ utilization of social networks. Motivation and engagement are crucial for academic achievement, and social media can provide a platform for connecting with peers, sharing information, and participating in discussions, thereby enhancing motivation and engagement (Song & Bonk, 2016; Ryan & Deci, 2022). Additionally, social media can facilitate a sense of community and belonging, motivating students to actively engage in their studies. Furthermore, strong academic habits, such as goal-setting and time management skills, are associated with social media use for academic purposes (Garrin, 2014). It is important to acknowledge that while this study did not find negative effects of social media, further research should explore the potential risks and benefits and consider contextual factors that may influence outcomes (Wong-Lo et al., 2011; Allcott & Gentzkow, 2017). Overall, these findings underscore the importance of fostering motivation, engagement, and strong academic habits to effectively leverage social media for educational purposes.
Previous studies have yielded mixed results regarding the relationship between social media and academic motivation and engagement. While some studies have found positive effects, others have reported negative effects or no significant effects (Lee, 2020; Skinner et al., 2022). The impact of social media on academic motivation and engagement is complex and multifaceted, and more research is needed to understand how to leverage social media effectively in educational contexts. Social media can be a source of stress and anxiety, particularly when it is used for social comparison or when negative feedback is received (Lee, 2020). Overall, the role of social media in academic motivation and engagement is complex and multifaceted (Skinner et al., 2022). It can have both positive and negative effects, depending on how it is used and the context in which it is used. Further research is needed to better understand the factors that influence the impact of social media on academic motivation and engagement, and to develop effective strategies for leveraging its potential benefits while mitigating its potential risks (Greenhow & Lewin, 2016).
There are also potential negative effects associated with social media use in the context of academic motivation and engagement, such as distraction and procrastination, which can reduce students’ motivation and engagement in academic tasks (Anierobi et al., 2021). Several studies provide insights into this relationship. For example, Kirschner and Karpinski (2010) found that students who used Facebook while studying had lower levels of academic achievement, while Junco (2012) discovered that students who used Twitter as part of their course had higher levels of engagement and academic success. Similarly, Manca and Ranieri (2016) found that students who used social media for educational purposes had higher levels of engagement, motivation, and satisfaction. Additionally, Roblyer, McDaniel, Webb, Herman, and Witty (2010) found that students who incorporated social media in their courses had higher levels of engagement, motivation, and satisfaction. Wang, Chen, and Liang (2011) reported that social media use had a positive impact on students’ academic achievement by facilitating knowledge sharing and easier access to learning materials. Kaplan and Haenlein (2010) proposed that social media could enhance student motivation and engagement by supporting self-determination theory principles.
The research findings have several implications for educational practice. Firstly, educators and institutions should recognize the positive relationship between motivation and students’ use of social networks for learning. They can leverage social media platforms to enhance student motivation and engagement by encouraging peer interaction, facilitating information sharing, and promoting active participation in discussions. Incorporating social media into instructional strategies can create a sense of community and belonging, fostering a supportive learning environment.
Secondly, the study highlights the importance of academic engagement in promoting the utilization of social networks for learning. Educators can encourage and promote active student engagement by integrating social media tools into their teaching practices. Creating online discussion forums, sharing relevant articles and videos, and organizing virtual study groups through social media can enhance student engagement and promote collaborative learning.
Furthermore, the findings emphasize the significance of cultivating effective study habits among students. Educators can incorporate explicit instruction and guidance on time management, goal-setting, note-taking, and other study skills to support students in developing strong academic habits. By promoting these habits, students are more likely to utilize social media platforms in a purposeful and productive manner for educational purposes.
LIMITATIONS
This study has several limitations that should be considered. Firstly, the use of intentional sampling may limit the generalizability of the findings. The sample size of 200 participants may not fully represent the diverse population of students, potentially leading to biased results and limiting the ability to draw broad conclusions. Additionally, the R-squared value of the regression model, which indicates the proportion of variance explained by the model, is 0.360. This suggests that there are other factors not accounted for in the study that may contribute to students’ use of social networks in learning. Therefore, caution should be exercised in interpreting the results and applying them to a wider context. Future research with larger and more diverse samples is needed to enhance the generalizability and strengthen the predictive power of the model.
REFERENCES
- Abbas, J., Aman, J., Nurunnabi, M., & Bano, S. (2019). The impact of social media on learning behavior for sustainable education: Evidence of students from selected universities in Pakistan. Sustainability, 11(6), 1683.
- Abdulahi, A., Samadi, B., & Gharleghi, B. (2014). A study on the negative effects of social networking sites such as Facebook among Asia Pacific University scholars in Malaysia. International Journal of Business and Social Science, 5(10).
- Agresti, A., & Finlay, B. (2009). Statistical methods for the social sciences(Vol. 207). Upper Saddle River, NJ: Pearson Prentice Hall.
- Akbari, E., Naderi, A., Simons, R. J., & Pilot, A. (2016). Student engagement and foreign language learning through online social networks. Asian-Pacific Journal of Second and Foreign Language Education, 1, 1-22.
- Akram, W., & Kumar, R. (2017). A study on positive and negative effects of social media on society. International Journal of Computer Sciences and Engineering, 5(10), 351-354.
- Allcott, H., & Gentzkow, M. (2017). Social media and fake news in the 2016 election. Journal of economic perspectives, 31(2), 211-236.
- Anierobi, E. I., Etodike, C. E., Anierobi, E. I., Okeke, N. U., & Ezennaka, A. O. (2021). Social media addiction as correlates of academic procrastination and achievement among undergraduates of Nnamdi Azikiwe University Awka, Nigeria. International Journal of Academic Research in Progressive Education and Development, 10(3), 20-33.
- Bandura, A. (2001). Social cognitive theory: An agentic perspective. Annual review of psychology, 52(1), 1-26.
- Bertot, J. C., Jaeger, P. T., & Hansen, D. (2012). The impact of polices on government social media usage: Issues, challenges, and recommendations. Government information quarterly, 29(1), 30-40.
- Blankenship, M. (2011). How social media can and should impact higher education. Education Digest, 76(7), 39-42.
- Bonk, C. J., Lee, M. M., Kou, X., Xu, S., & Sheu, F. R. (2015). Understanding the self-directed online learning preferences, goals, achievements, and challenges of MIT OpenCourseWare subscribers. Journal of Educational Technology & Society, 18(2), 349-368.
- Boyd, D. M., & Ellison, N. B. (2007). Social network sites: Definition, history, and scholarship. Journal of computer‐mediated Communication, 13(1), 210-230.
- Bruns, A., & Bahnisch, M. (2009). Social media: Tools for user-generated content: Social drivers behind growing consumer participation in user-led content generation, Volume 1-State of the art.
- Chien, N. B., & Thanh, N. N. (2022). The impact of good governance on the people’s satisfaction with public administrative services in Vietnam. Administrative Sciences, 12(1), 35.
- Chuang, H. H. (2016). Leveraging CRT awareness in creating web-based projects through use of online collaborative learning for pre-service teachers. Educational Technology Research and Development, 64, 857-876.
- Cook, D. A., & Artino Jr, A. R. (2016). Motivation to learn: an overview of contemporary theories. Medical education, 50(10), 997-1014.
- Cortina, J. M. (1993). What is coefficient alpha? An examination of theory and applications. Journal of applied psychology, 78(1), 98.
- Couldry, N. (2012). Media, society, world: Social theory and digital media practice. Polity.
- Eccles, J., & Wang, M. T. (2012). Part I commentary: So what is student engagement anyway?. Handbook of research on student engagement, 133-145.
- Fabrigar, L. R., Wegener, D. T., MacCallum, R. C., & Strahan, E. J. (1999). Evaluating the use of exploratory factor analysis in psychological research. Psychological methods, 4(3), 272.
- Fidell, S., Tabachnick, B., Mestre, V., & Fidell, L. (2013). Aircraft noise-induced awakenings are more reasonably predicted from relative than from absolute sound exposure levels. The Journal of the Acoustical Society of America, 134(5), 3645-3653.
- Filgona, J., Sakiyo, J., Gwany, D. M., & Okoronka, A. U. (2020). Motivation in learning. Asian Journal of Education and social studies, 10(4), 16-37.
- Fornell, C., & Larcker, D. F. (1981). Structural equation models with unobservable variables and measurement error: Algebra and statistics.
- Garrin, J. M. (2014). Self-efficacy, self-determination, and self-regulation: The role of the fitness professional in social change agency. Journal of Sustainable Social Change, 6(1), 4.
- Gorsuch, R. L. (1990). Common factor analysis versus component analysis: Some well and little known facts. Multivariate behavioral research, 25(1), 33-39.
- Graue, C. (2015). Qualitative data analysis. International Journal of Sales, Retailing & Marketing, 4(9), 5-14.
- Greene, W. H., & Hensher, D. A. (2003). A latent class model for discrete choice analysis: contrasts with mixed logit. Transportation Research Part B: Methodological, 37(8), 681-698.
- Greenhow, C., & Lewin, C. (2016). Social media and education: Reconceptualizing the boundaries of formal and informal learning. Learning, media and technology, 41(1), 6-30.
- Gulzar, M. A., Ahmad, M., Hassan, M., & Rasheed, M. I. (2022). How social media use is related to student engagement and creativity: investigating through the lens of intrinsic motivation. Behaviour & Information Technology, 41(11), 2283-2293.
- Hair, A. (1998). Tatham, and Black. Análisis multivariante.
- Hsieh, T. L. (2014). Motivation matters? The relationship among different types of learning motivation, engagement behaviors and learning outcomes of undergraduate students in Taiwan. Higher Education, 68, 417-433.
- Hwang, M. Y., Hong, J. C., Hao, Y. W., & Jong, J. T. (2011). Elders’ usability, dependability, and flow experiences on embodied interactive video games. Educational Gerontology, 37(8), 715-731.
- Imlawi, J., Gregg, D., & Karimi, J. (2015). Student engagement in course-based social networks: The impact of instructor credibility and use of communication. Computers & Education, 88, 84-96.
- Jeong, K. O. (2019). Online collaborative language learning for enhancing learner motivation and classroom engagement. International Journal of Contents, 15(4), 89-96.
- Johnson, D. W. (1991). Cooperative Learning: Increasing College Faculty Instructional Productivity. ASHE-ERIC Higher Education Report No. 4, 1991. ASHE-ERIC Higher Education Reports, George Washington University, One Dupont Circle, Suite 630, Washington, DC 20036-1183.
- Junco, R. (2012). The relationship between frequency of Facebook use, participation in Facebook activities, and student engagement. Computers & education, 58(1), 162-171.
- Kalaian, H. A., & Raudenbush, S. W. (1996). A multivariate mixed linear model for meta-analysis. Psychological methods, 1(3), 227.
- Kaplan, A. M., & Haenlein, M. (2010). Users of the world, unite! The challenges and opportunities of Social Media. Business horizons, 53(1), 59-68.
- Kim, J. O., & Mueller, C. W. (1978). Factor analysis: Statistical methods and practical issues(Vol. 14). sage.
- Kirschner, P. A., & Karpinski, A. C. (2010). Facebook® and academic performance. Computers in human behavior, 26(6), 1237-1245.
- Kline, R. B. (2023). Principles and practice of structural equation modeling. Guilford publications.
- Lankshear, C., & Knobel, M. (2011). EBOOK: New Literacies: Everyday Practices and Social Learning. McGraw-Hill Education (UK).
- Lee, J. K. (2020). The effects of social comparison orientation on psychological well-being in social networking sites: Serial mediation of perceived social support and self-esteem. Current Psychology, 1-13.
- Luan, N. T., & Thanh, N. N. (2022). The impact of competency factors on job performance: a survey of student affairs staff in Vietnam universities. PalArch’s Journal of Archaeology of Egypt/Egyptology, 19(1), 991-1012.
- Luszczynska, A., & Schwarzer, R. (2015). Social cognitive theory. Fac Health Sci Publ, 225-51.
- Madge, C., Meek, J., Wellens, J., & Hooley, T. (2009). Facebook, social integration and informal learning at university:‘It is more for socialising and talking to friends about work than for actually doing work’. Learning, media and technology, 34(2), 141-155.
- Manca, S., & Ranieri, M. (2016). Facebook and the others. Potentials and obstacles of social media for teaching in higher education. Computers & Education, 95, 216-230.
- Miller, M. D., Linn, R., & Gronlund, N. (2009). Measurement and evaluation in teaching.
- Nakamura, J., & Csikszentmihalyi, M. (2009). Flow theory and research. Handbook of positive psychology, 195, 206.
- Nghi, T. N., Thu, H. T., & Dinh, T. T. (2022). The Relationship between Public Service Motivation, Work Enjoyment, and Task Performance: A Preliminary study of Healthcare Workers in Vietnam. Journal of Liberty and International Affairs, 8(2), 47-60.
- Oh, J. E., Chan, Y. K., & Kim, K. V. (2020). Social Media and E-Portfolios: Impacting Design Students’ Motivation through Project-Based Learning. IAFOR Journal of Education, 8(3), 41-58.
- Osborne, J. W. (2000). Advantages of hierarchical linear modeling. Practical Assessment, Research, and Evaluation, 7(1), 1.
- Owusu-Acheaw, M., & Larson, A. G. (2015). Use of social media and its impact on academic performance of tertiary institution students: A study of students of Koforidua Polytechnic, Ghana. Journal of Education and Practice, 6(6), 94-101.
- Pempek, T. A., Yermolayeva, Y. A., & Calvert, S. L. (2009). College students’ social networking experiences on Facebook. Journal of applied developmental psychology, 30(3), 227-238.
- Roblyer, M. D., McDaniel, M., Webb, M., Herman, J., & Witty, J. V. (2010). Findings on Facebook in higher education: A comparison of college faculty and student uses and perceptions of social networking sites. The Internet and higher education, 13(3), 134-140.
- Roblyer, M. D., McDaniel, M., Webb, M., Herman, J., & Witty, J. V. (2010). Findings on Facebook in higher education: A comparison of college faculty and student uses and perceptions of social networking sites. The Internet and higher education, 13(3), 134-140.
- Ryan, R. M., & Deci, E. L. (2000). Intrinsic and extrinsic motivations: Classic definitions and new directions. Contemporary educational psychology, 25(1), 54-67.
- Ryan, R. M., & Deci, E. L. (2022). Self-determination theory. In Encyclopedia of quality of life and well-being research(pp. 1-7). Cham: Springer International Publishing.
- Schroeder, A., Minocha, S., & Schneider, C. (2010). The strengths, weaknesses, opportunities and threats of using social software in higher and further education teaching and learning. Journal of computer assisted learning, 26(3), 159-174.
- Skinner, E. A., Kindermann, T. A., Vollet, J. W., & Rickert, N. P. (2022). Complex Social Ecologies and the Development of Academic Motivation. Educational Psychology Review, 1-37.
- Song, D., & Bonk, C. J. (2016). Motivational factors in self-directed informal learning from online learning resources. Cogent Education, 3(1), 1205838.
- Thanh, N. N., Thuy, H. T. T., Hoa, N. T. T., & Thien, N. D. (2022). The relationship between ethical leadership, job engagement, and job performance: evidence from the public sector in Vietnam. PalArch’s Journal of Archaeology of Egypt/Egyptology, 19(2), 1161-1176.
- Thanh, N. N., Thuy, N. T., Thao, B. T., & Huong, H. T. T. (2023). Policies for Religious and the Practice of Burning Votive Papers for the deceased: a survey in vietnam. Synesis (ISSN 1984-6754), 15(2), 208-222.
- Thanh, N. N., Tung, P. H., Thu, N. H., Kien, P. D., & Nguyet, N. A. (2021). Factors affecting the share of fake news about covid-19 outbreak on social networks in vietnam. Liberty & Int’l Aff., 7, 179.
- Tung, P. H., Thanh, N. N., Hang, T. T. T., & Thuy, N. T. (2023). Policy on Foreign Direct Investment Attraction of Vietnam in the Context of Global Foreign Direct Investment Movement. Synesis (ISSN 1984-6754), 15(1), 195-219.
- Wang, Q., Chen, W., & Liang, Y. (2011). The Efects of Social Media on College Students.https://scholarsarchive.jwu.edu
- Wankel, C. (2012). Educating educators with social media. Development and Learning in Organizations: An International Journal, 26(3).
- Wigfield, A. (1994). Expectancy-value theory of achievement motivation: A developmental perspective. Educational psychology review, 6, 49-78.
- Wong-Lo, M., Bullock, L. M., & Gable, R. A. (2011). Cyber bullying: Practices to face digital aggression. Emotional and Behavioural Difficulties, 16(3), 317-325.
Appendix
QUESTIONNAIRE
Your profile: Please select ONE answer from each statement that best describes you.
Your age:…………………….
Your gender:☐male ☐female
Year in College:☐1 year ☐2 years ☐3 years ☐4 years ☐over 4 years
What is your current academic standing?
☐freshman ☐sophomore☐junior☐senior
What is your major area of study? ………………………………………
What is your current GPA? …………………………………………….
☐< 2.0 ☐2.0-2.5 ☐2.5-3.0 ☐3.0-3.5☐3.5-4.0
Instead, mark the number that best represents your viewpoint on each survey topic on a scale of 1 to 5, as shown.
Media_Use | Social Media Use | |||||
Media_Use1 | How often do you use social media for academic purposes? | ☐ | ☐ | ☐ | ☐ | ☐ |
Media_Use2 | How often do you use social media to communicate with classmates about coursework? | ☐ | ☐ | ☐ | ☐ | ☐ |
Media_Use3 | How often do you use social media to connect with professors outside of class? | ☐ | ☐ | ☐ | ☐ | ☐ |
Media_Use4 | How often do you use social media to research academic topics? | ☐ | ☐ | ☐ | ☐ | ☐ |
Motivation | Academic Motivation | |||||
Motivation1 | I feel motivated to do well in my courses. | ☐ | ☐ | ☐ | ☐ | ☐ |
Motivation2 | I am interested in the subject matter of my courses. | ☐ | ☐ | ☐ | ☐ | ☐ |
Motivation3 | I set challenging goals for myself in my academic work. | ☐ | ☐ | ☐ | ☐ | ☐ |
Motivation4 | I am willing to put in the effort necessary to succeed academically. | ☐ | ☐ | ☐ | ☐ | ☐ |
Engagement | Academic Engagement | |||||
Engagement1 | I actively participate in class discussions. | ☐ | ☐ | ☐ | ☐ | ☐ |
Engagement2 | I am able to apply what I learn in my courses to real-world situations. | ☐ | ☐ | ☐ | ☐ | ☐ |
Engagement3 | I seek out additional resources to enhance my learning. | ☐ | ☐ | ☐ | ☐ | ☐ |
Engagement4 | I am curious about the subjects I am studying. | ☐ | ☐ | ☐ | ☐ | ☐ |
Habits | Study Habits | |||||
Habits1 | I manage my time effectively to complete coursework. | ☐ | ☐ | ☐ | ☐ | ☐ |
Habits2 | I review course material regularly to reinforce my learning. | ☐ | ☐ | ☐ | ☐ | ☐ |
Habits3 | I take notes during lectures and while studying. | ☐ | ☐ | ☐ | ☐ | ☐ |
Habits4 | I ask questions when I don’t understand something. | ☐ | ☐ | ☐ | ☐ | ☐ |
Thank you for your participation.
The Role of Social Media in Academic Motivation and Engagement: A Case Study of Undergraduate Students in Vitenam
Nguyen Thi Quynh Trang
National Academy of Public Administration, Vietnam
Vol 3 No 7 (2023): Volume 03 Issue 07 July 2023
Article Date Published : 25 July 2023 | Page No.: 1423-1434
Abstract :
This study examines the impact of social media on academic motivation and engagement among undergraduate students in Vietnam. Regression analysis reveals that motivation, engagement, and study habits influence students’ use of social networks for learning. Motivation shows a positive and significant relationship with media use, supporting previous research emphasizing its role in academic success. Social media platforms facilitate peer connections, information sharing, and participation in discussions, enhancing student motivation. Engagement also exhibits a positive association with social media use, highlighting its importance in leveraging these platforms for learning. Furthermore, study habits demonstrate a positive link with social media use, indicating that effective habits contribute to academic success and the utilization of social media for educational purposes. Importantly, this study does not find evidence of negative effects associated with social networks, although they can distract from academic pursuits when used non-academically. These findings underscore the significance of motivation, engagement, and study habits in effectively harnessing social networks for educational purposes, providing insights for educational practitioners and policymakers in promoting positive technology integration in learning environments.
Keywords :
Social media; Academic motivation; Academic engagement; Undergraduate students; Case study; VietnamReferences :
- Abbas, J., Aman, J., Nurunnabi, M., & Bano, S. (2019). The impact of social media on learning behavior for sustainable education: Evidence of students from selected universities in Pakistan. Sustainability, 11(6), 1683.
- Abdulahi, A., Samadi, B., & Gharleghi, B. (2014). A study on the negative effects of social networking sites such as Facebook among Asia Pacific University scholars in Malaysia. International Journal of Business and Social Science, 5(10).
- Agresti, A., & Finlay, B. (2009). Statistical methods for the social sciences(Vol. 207). Upper Saddle River, NJ: Pearson Prentice Hall.
- Akbari, E., Naderi, A., Simons, R. J., & Pilot, A. (2016). Student engagement and foreign language learning through online social networks. Asian-Pacific Journal of Second and Foreign Language Education, 1, 1-22.
- Akram, W., & Kumar, R. (2017). A study on positive and negative effects of social media on society. International Journal of Computer Sciences and Engineering, 5(10), 351-354.
- Allcott, H., & Gentzkow, M. (2017). Social media and fake news in the 2016 election. Journal of economic perspectives, 31(2), 211-236.
- Anierobi, E. I., Etodike, C. E., Anierobi, E. I., Okeke, N. U., & Ezennaka, A. O. (2021). Social media addiction as correlates of academic procrastination and achievement among undergraduates of Nnamdi Azikiwe University Awka, Nigeria. International Journal of Academic Research in Progressive Education and Development, 10(3), 20-33.
- Bandura, A. (2001). Social cognitive theory: An agentic perspective. Annual review of psychology, 52(1), 1-26.
- Bertot, J. C., Jaeger, P. T., & Hansen, D. (2012). The impact of polices on government social media usage: Issues, challenges, and recommendations. Government information quarterly, 29(1), 30-40.
- Blankenship, M. (2011). How social media can and should impact higher education. Education Digest, 76(7), 39-42.
- Bonk, C. J., Lee, M. M., Kou, X., Xu, S., & Sheu, F. R. (2015). Understanding the self-directed online learning preferences, goals, achievements, and challenges of MIT OpenCourseWare subscribers. Journal of Educational Technology & Society, 18(2), 349-368.
- Boyd, D. M., & Ellison, N. B. (2007). Social network sites: Definition, history, and scholarship. Journal of computer‐mediated Communication, 13(1), 210-230.
- Bruns, A., & Bahnisch, M. (2009). Social media: Tools for user-generated content: Social drivers behind growing consumer participation in user-led content generation, Volume 1-State of the art.
- Chien, N. B., & Thanh, N. N. (2022). The impact of good governance on the people’s satisfaction with public administrative services in Vietnam. Administrative Sciences, 12(1), 35.
- Chuang, H. H. (2016). Leveraging CRT awareness in creating web-based projects through use of online collaborative learning for pre-service teachers. Educational Technology Research and Development, 64, 857-876.
- Cook, D. A., & Artino Jr, A. R. (2016). Motivation to learn: an overview of contemporary theories. Medical education, 50(10), 997-1014.
- Cortina, J. M. (1993). What is coefficient alpha? An examination of theory and applications. Journal of applied psychology, 78(1), 98.
- Couldry, N. (2012). Media, society, world: Social theory and digital media practice. Polity.
- Eccles, J., & Wang, M. T. (2012). Part I commentary: So what is student engagement anyway?. Handbook of research on student engagement, 133-145.
- Fabrigar, L. R., Wegener, D. T., MacCallum, R. C., & Strahan, E. J. (1999). Evaluating the use of exploratory factor analysis in psychological research. Psychological methods, 4(3), 272.
- Fidell, S., Tabachnick, B., Mestre, V., & Fidell, L. (2013). Aircraft noise-induced awakenings are more reasonably predicted from relative than from absolute sound exposure levels. The Journal of the Acoustical Society of America, 134(5), 3645-3653.
- Filgona, J., Sakiyo, J., Gwany, D. M., & Okoronka, A. U. (2020). Motivation in learning. Asian Journal of Education and social studies, 10(4), 16-37.
- Fornell, C., & Larcker, D. F. (1981). Structural equation models with unobservable variables and measurement error: Algebra and statistics.
- Garrin, J. M. (2014). Self-efficacy, self-determination, and self-regulation: The role of the fitness professional in social change agency. Journal of Sustainable Social Change, 6(1), 4.
- Gorsuch, R. L. (1990). Common factor analysis versus component analysis: Some well and little known facts. Multivariate behavioral research, 25(1), 33-39.
- Graue, C. (2015). Qualitative data analysis. International Journal of Sales, Retailing & Marketing, 4(9), 5-14.
- Greene, W. H., & Hensher, D. A. (2003). A latent class model for discrete choice analysis: contrasts with mixed logit. Transportation Research Part B: Methodological, 37(8), 681-698.
- Greenhow, C., & Lewin, C. (2016). Social media and education: Reconceptualizing the boundaries of formal and informal learning. Learning, media and technology, 41(1), 6-30.
- Gulzar, M. A., Ahmad, M., Hassan, M., & Rasheed, M. I. (2022). How social media use is related to student engagement and creativity: investigating through the lens of intrinsic motivation. Behaviour & Information Technology, 41(11), 2283-2293.
- Hair, A. (1998). Tatham, and Black. Análisis multivariante.
- Hsieh, T. L. (2014). Motivation matters? The relationship among different types of learning motivation, engagement behaviors and learning outcomes of undergraduate students in Taiwan. Higher Education, 68, 417-433.
- Hwang, M. Y., Hong, J. C., Hao, Y. W., & Jong, J. T. (2011). Elders’ usability, dependability, and flow experiences on embodied interactive video games. Educational Gerontology, 37(8), 715-731.
- Imlawi, J., Gregg, D., & Karimi, J. (2015). Student engagement in course-based social networks: The impact of instructor credibility and use of communication. Computers & Education, 88, 84-96.
- Jeong, K. O. (2019). Online collaborative language learning for enhancing learner motivation and classroom engagement. International Journal of Contents, 15(4), 89-96.
- Johnson, D. W. (1991). Cooperative Learning: Increasing College Faculty Instructional Productivity. ASHE-ERIC Higher Education Report No. 4, 1991. ASHE-ERIC Higher Education Reports, George Washington University, One Dupont Circle, Suite 630, Washington, DC 20036-1183.
- Junco, R. (2012). The relationship between frequency of Facebook use, participation in Facebook activities, and student engagement. Computers & education, 58(1), 162-171.
- Kalaian, H. A., & Raudenbush, S. W. (1996). A multivariate mixed linear model for meta-analysis. Psychological methods, 1(3), 227.
- Kaplan, A. M., & Haenlein, M. (2010). Users of the world, unite! The challenges and opportunities of Social Media. Business horizons, 53(1), 59-68.
- Kim, J. O., & Mueller, C. W. (1978). Factor analysis: Statistical methods and practical issues(Vol. 14). sage.
- Kirschner, P. A., & Karpinski, A. C. (2010). Facebook® and academic performance. Computers in human behavior, 26(6), 1237-1245.
- Kline, R. B. (2023). Principles and practice of structural equation modeling. Guilford publications.
- Lankshear, C., & Knobel, M. (2011). EBOOK: New Literacies: Everyday Practices and Social Learning. McGraw-Hill Education (UK).
- Lee, J. K. (2020). The effects of social comparison orientation on psychological well-being in social networking sites: Serial mediation of perceived social support and self-esteem. Current Psychology, 1-13.
- Luan, N. T., & Thanh, N. N. (2022). The impact of competency factors on job performance: a survey of student affairs staff in Vietnam universities. PalArch’s Journal of Archaeology of Egypt/Egyptology, 19(1), 991-1012.
- Luszczynska, A., & Schwarzer, R. (2015). Social cognitive theory. Fac Health Sci Publ, 225-51.
- Madge, C., Meek, J., Wellens, J., & Hooley, T. (2009). Facebook, social integration and informal learning at university:‘It is more for socialising and talking to friends about work than for actually doing work’. Learning, media and technology, 34(2), 141-155.
- Manca, S., & Ranieri, M. (2016). Facebook and the others. Potentials and obstacles of social media for teaching in higher education. Computers & Education, 95, 216-230.
- Miller, M. D., Linn, R., & Gronlund, N. (2009). Measurement and evaluation in teaching.
- Nakamura, J., & Csikszentmihalyi, M. (2009). Flow theory and research. Handbook of positive psychology, 195, 206.
- Nghi, T. N., Thu, H. T., & Dinh, T. T. (2022). The Relationship between Public Service Motivation, Work Enjoyment, and Task Performance: A Preliminary study of Healthcare Workers in Vietnam. Journal of Liberty and International Affairs, 8(2), 47-60.
- Oh, J. E., Chan, Y. K., & Kim, K. V. (2020). Social Media and E-Portfolios: Impacting Design Students’ Motivation through Project-Based Learning. IAFOR Journal of Education, 8(3), 41-58.
- Osborne, J. W. (2000). Advantages of hierarchical linear modeling. Practical Assessment, Research, and Evaluation, 7(1), 1.
- Owusu-Acheaw, M., & Larson, A. G. (2015). Use of social media and its impact on academic performance of tertiary institution students: A study of students of Koforidua Polytechnic, Ghana. Journal of Education and Practice, 6(6), 94-101.
- Pempek, T. A., Yermolayeva, Y. A., & Calvert, S. L. (2009). College students’ social networking experiences on Facebook. Journal of applied developmental psychology, 30(3), 227-238.
- Roblyer, M. D., McDaniel, M., Webb, M., Herman, J., & Witty, J. V. (2010). Findings on Facebook in higher education: A comparison of college faculty and student uses and perceptions of social networking sites. The Internet and higher education, 13(3), 134-140.
- Roblyer, M. D., McDaniel, M., Webb, M., Herman, J., & Witty, J. V. (2010). Findings on Facebook in higher education: A comparison of college faculty and student uses and perceptions of social networking sites. The Internet and higher education, 13(3), 134-140.
- Ryan, R. M., & Deci, E. L. (2000). Intrinsic and extrinsic motivations: Classic definitions and new directions. Contemporary educational psychology, 25(1), 54-67.
- Ryan, R. M., & Deci, E. L. (2022). Self-determination theory. In Encyclopedia of quality of life and well-being research(pp. 1-7). Cham: Springer International Publishing.
- Schroeder, A., Minocha, S., & Schneider, C. (2010). The strengths, weaknesses, opportunities and threats of using social software in higher and further education teaching and learning. Journal of computer assisted learning, 26(3), 159-174.
- Skinner, E. A., Kindermann, T. A., Vollet, J. W., & Rickert, N. P. (2022). Complex Social Ecologies and the Development of Academic Motivation. Educational Psychology Review, 1-37.
- Song, D., & Bonk, C. J. (2016). Motivational factors in self-directed informal learning from online learning resources. Cogent Education, 3(1), 1205838.
- Thanh, N. N., Thuy, H. T. T., Hoa, N. T. T., & Thien, N. D. (2022). The relationship between ethical leadership, job engagement, and job performance: evidence from the public sector in Vietnam. PalArch’s Journal of Archaeology of Egypt/Egyptology, 19(2), 1161-1176.
- Thanh, N. N., Thuy, N. T., Thao, B. T., & Huong, H. T. T. (2023). Policies for Religious and the Practice of Burning Votive Papers for the deceased: a survey in vietnam. Synesis (ISSN 1984-6754), 15(2), 208-222.
- Thanh, N. N., Tung, P. H., Thu, N. H., Kien, P. D., & Nguyet, N. A. (2021). Factors affecting the share of fake news about covid-19 outbreak on social networks in vietnam. Liberty & Int’l Aff., 7, 179.
- Tung, P. H., Thanh, N. N., Hang, T. T. T., & Thuy, N. T. (2023). Policy on Foreign Direct Investment Attraction of Vietnam in the Context of Global Foreign Direct Investment Movement. Synesis (ISSN 1984-6754), 15(1), 195-219.
- Wang, Q., Chen, W., & Liang, Y. (2011). The Efects of Social Media on College Students.https://scholarsarchive.jwu.edu
- Wankel, C. (2012). Educating educators with social media. Development and Learning in Organizations: An International Journal, 26(3).
- Wigfield, A. (1994). Expectancy-value theory of achievement motivation: A developmental perspective. Educational psychology review, 6, 49-78.
- Wong-Lo, M., Bullock, L. M., & Gable, R. A. (2011). Cyber bullying: Practices to face digital aggression. Emotional and Behavioural Difficulties, 16(3), 317-325.
Author's Affiliation
Nguyen Thi Quynh Trang
National Academy of Public Administration, Vietnam
Article Details
- Issue: Vol 3 No 7 (2023): Volume 03 Issue 07 July 2023
- Page No.: 1423-1434
- Published : 25 July 2023
- DOI: https://doi.org/10.55677/ijssers/V03I7Y2023-32
How to Cite :
The Role of Social Media in Academic Motivation and Engagement: A Case Study of Undergraduate Students in Vitenam. Nguyen Thi Quynh Trang, 3(7), 1423-1434. Retrieved from https://ijssers.org/single-view/?id=8623&pid=8472
HTML format
0
View
377
Copyrights & License
This work is licenced under a Creative Commons Attribution 4.0 International License.
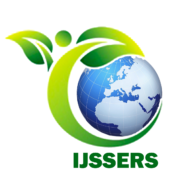
International Journal of Social Science and Education Research Studies
Main Menu
